A Leader in three Gartner® Magic Quadrant™ reports
Informatica is named a Leader in the Gartner® Magic Quadrant™ for Data Integration Tools, Gartner® Magic Quadrant™ for iPaaS and Gartner® Magic Quadrant™ for Data Quality Solutions
Data chaos becomes
data clarity with Informatica
We empower businesses to realize transformative outcomes by bringing their data and AI to life. When properly unlocked, data becomes a living and trusted asset that's democratized across the organization. Through our AI-powered Intelligent Data Management Cloud™, companies are driving better business results and creating a competitive advantage.
Is your data ready for AI?
Take 2 minutes to answer these 5 questions
and get a free custom report to help build your strategy.
Do you want to trust your data to automate processes, enable AI and analytics?
Would you like to streamline business processes, analytics, and AI across multiple applications?
Would you like to break down data silos and create an enriched 360° view of your customers?
Would you like to automate thousands of data management tasks without integrating multiple technologies?
Do you want to invest in one use case and be able to leverage that same investment for multiple use cases?
Take these steps to make your data ready for AI
Benefits of the Intelligent Data Management Cloud
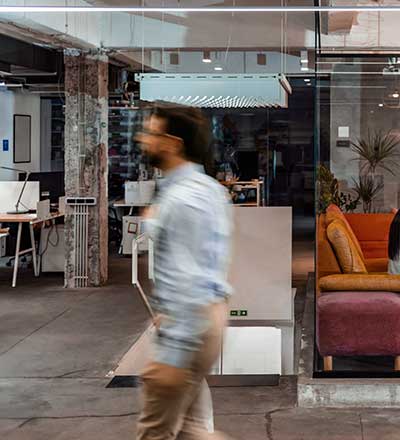
Open and Flexible
Built on industry standards, this cloud platform works with any reference architecture
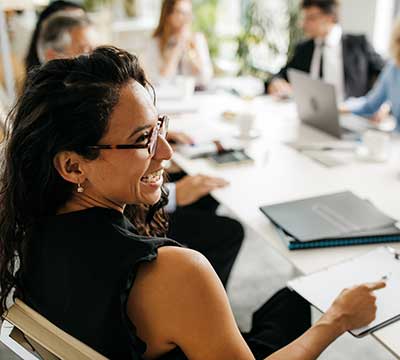
Multi-cloud and Hybrid
Supports the cloud model that best suits your business for migrating on-premises data
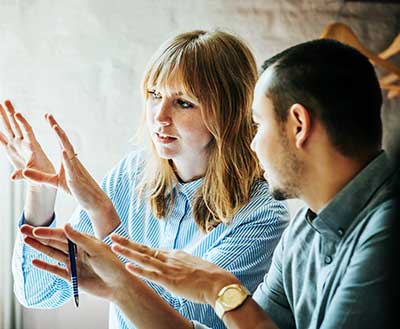
Low Code, No Code
Avoids the risk of custom coding with the power of AI and machine learning in one solution
Where data and AI do more:
Informatica Intelligent Data Management Cloud
Powered by CLAIRE®, our AI engine, it’s the only cloud dedicated to managing data of any type, pattern, complexity, or workload across any location—all on a single platform with a simple and flexible consumption-based pricing model.

data consumers
Intelligent Data Management Cloud
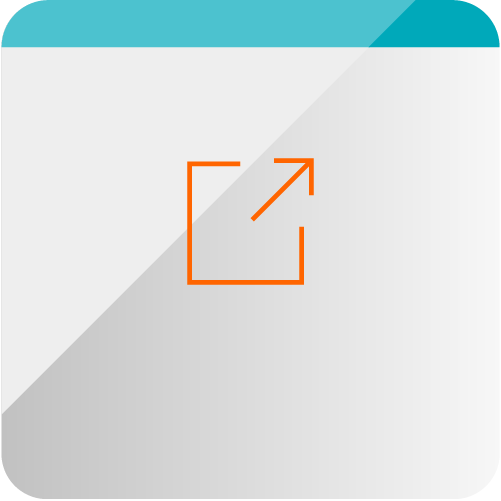
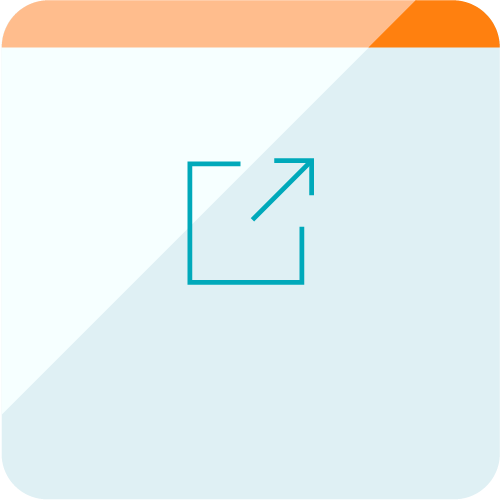
data integration & Engineering
Access and integrate data at scale using elastic and serverless computing.
LEARN MORE
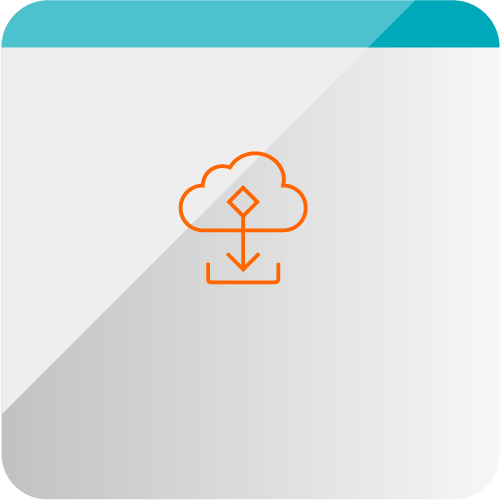
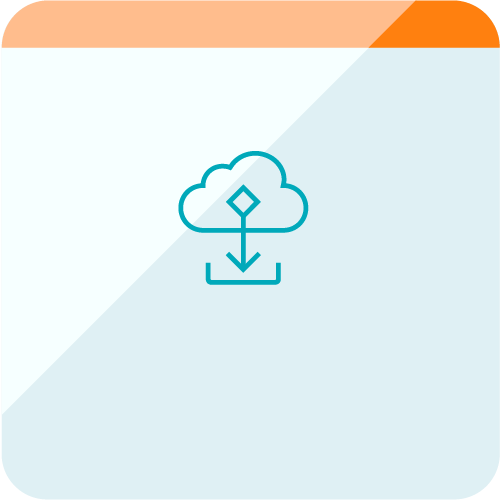
API & App
Integration
Connect your applications and automate end-to-end business processes.
LEARN MORE
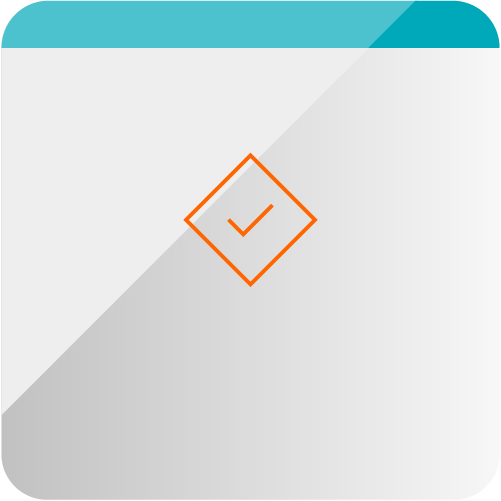
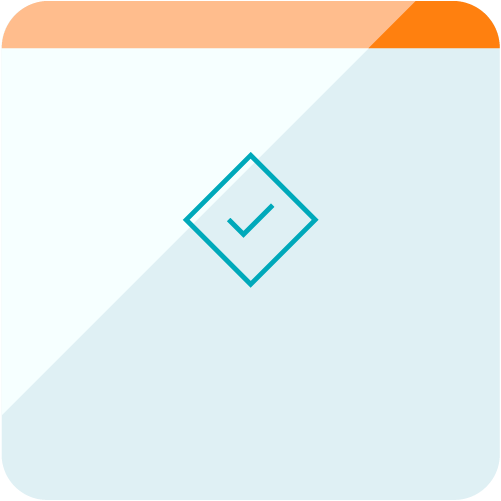
data quality & observability
Deliver consistent, trusted, and governed data across the enterprise.
LEARN MORE
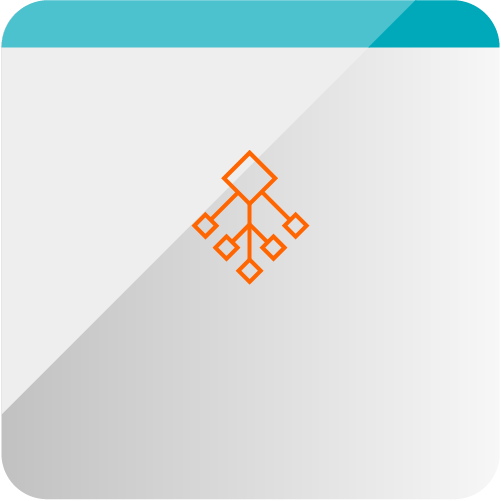
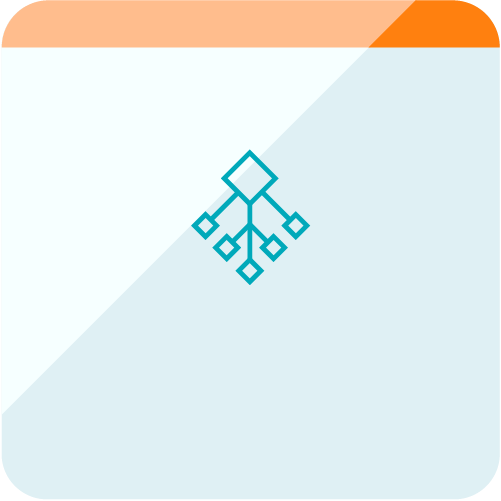
MDM & 360
Applications
Innovate with 360 views of business data and trusted intelligent insights.
LEARN
MORE

DATA SOURCES
Drive better AI
with better data
Deliver trusted data for
analytics and AI
Let CLAIRE do
the heavy lifting
A Leader in three Gartner® Magic Quadrant™ reports
Recognized for Data Integration, Data Quality and Master Data Management* (MDM)
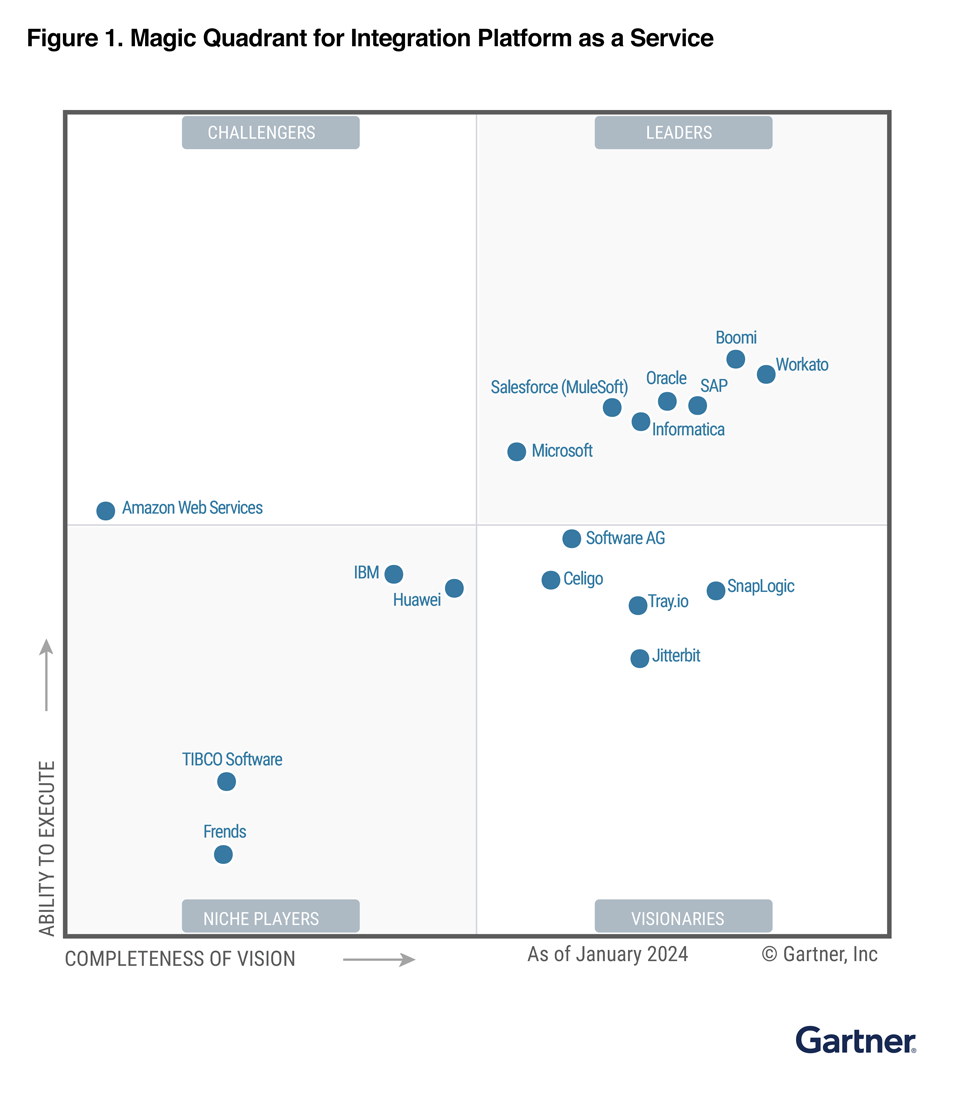
Boost your integration efficiency and AI-driven automation.
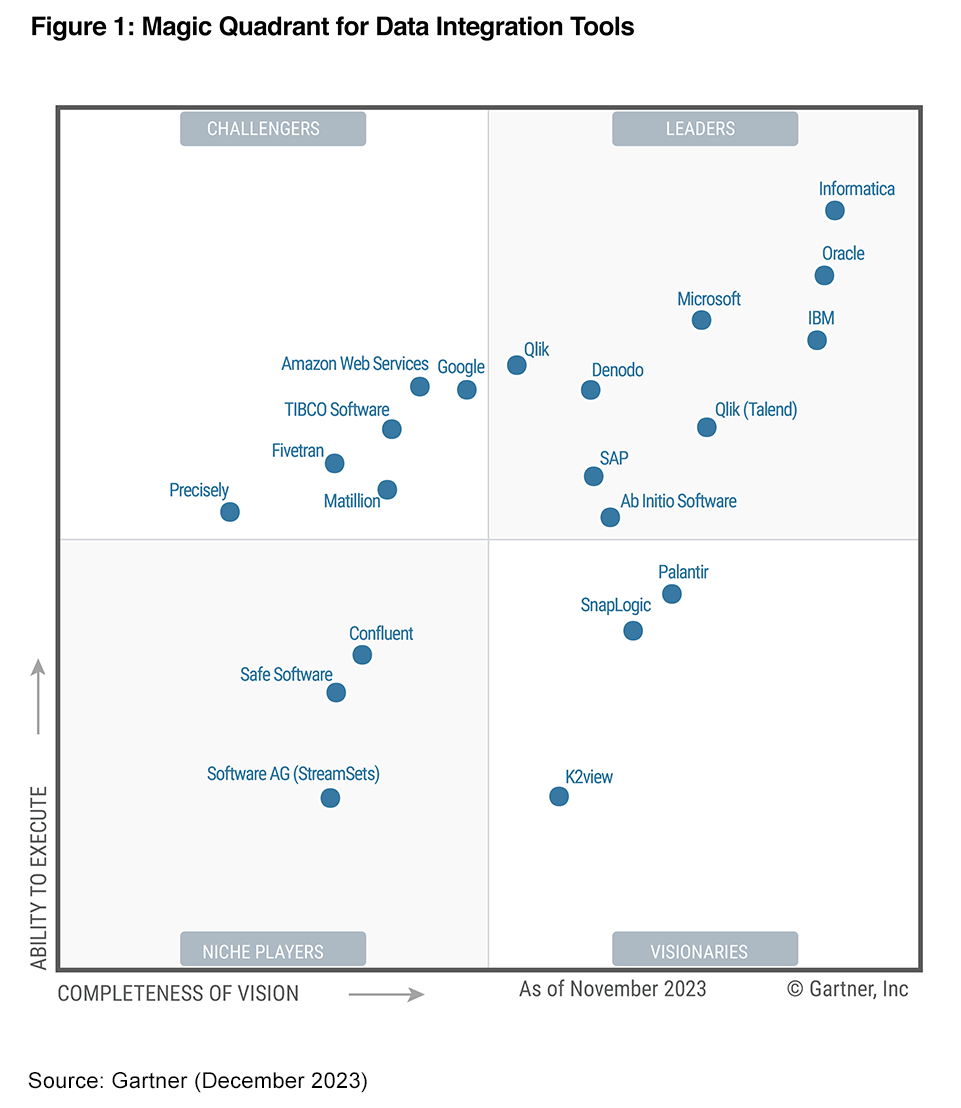
Orchestrate, unify, govern, and share your data.
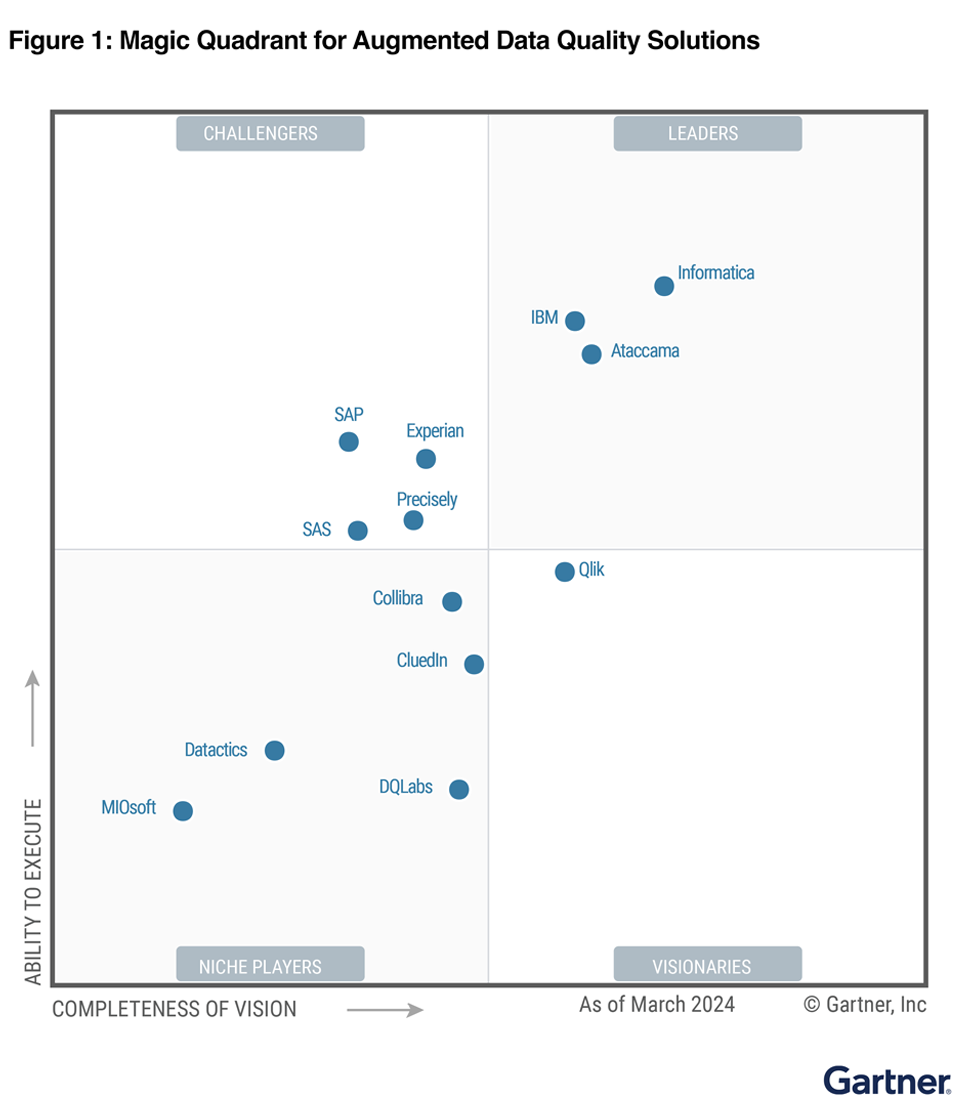
Accelerate key programs and fuel your business growth.
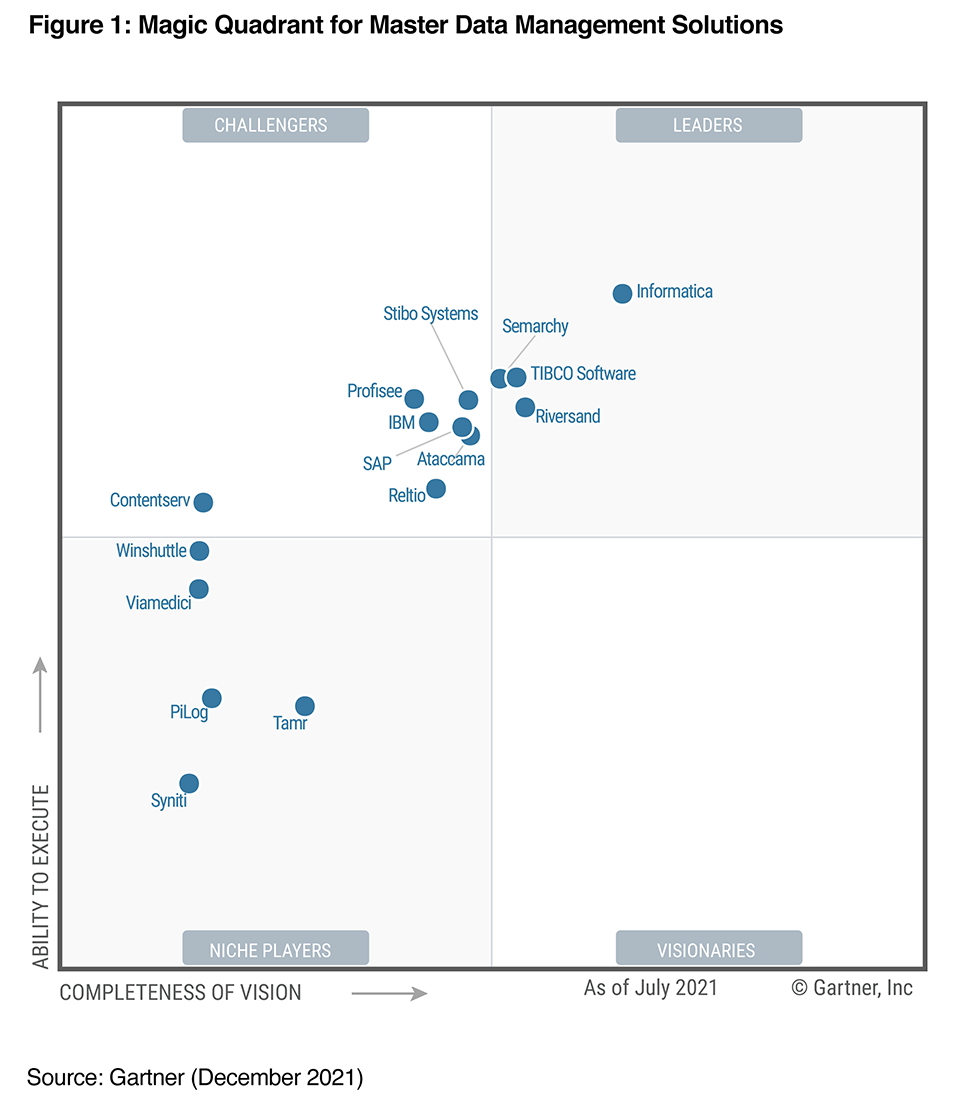
Deliver trusted, connected and contextual master data.
*Informatica is a Leader in the 2024 Magic Quadrant for iPaaS, 2023 Magic Quadrant for Data Integration, 2024 Magic Quadrant for Augmented Data Quality Solutions, and December 2021 Magic Quadrant for MDM.
Three ways to make your data do more
Office of the CDO
NEW Research – top priorities for 600 data leaders
Discover data strategies for implementing AI, reducing data complexity and delivering better business outcomes.
IT
Top data strategy best practices from IT leaders
Learn from top companies on how to build world-class data management capabilities to make better decisions.
SALES, MARKETING & DIGITAL Commerce
How to deliver exceptional customer experiences
Discover how intelligent data management will help enrich your customer’s experience and improve loyalty.