Explore how big data and data engineering can transform the manufacturing industry, both now and in the future
Why do manufacturers need big data solutions?
In the data-driven economy, turning data into actionable analytics is the best way to boost efficiency, quality, and productivity. That's as true on the shop floor as anywhere else – and maybe more so. Manufacturers of all types of products are integrating Internet of Things (IoT) technology and operationalizing the resulting streaming data to improve industrial processes
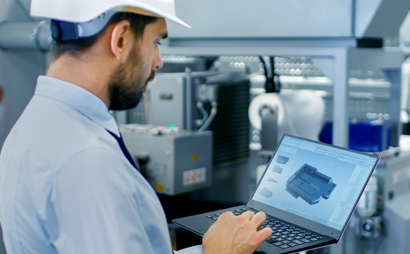
IoT gives manufacturers a new look into their processes and products, down to an extremely granular level of detail. The more IoT systems manufacturers adopt, the more real-time streaming data they need to manage. In addition to improving their ability to ingest, enrich, and cleanse big data to make sure they can trust it for both systems and analytics, they need to be able to apply artificial intelligence (AI) and machine learning (ML) to discover patterns and build models they can then operationalize with the necessary automation and scale.
Once they do so, the sky’s the limit. The insights gleaned from IoT and other high-volume, high-velocity data sources holds vast promise for revolutionizing the manufacturing industry in a way that lives up to the transformative implications of the term "Industry 4.0." Manufacturing big data use cases run the gamut from improved product development to optimizing spend. Let’s look at three compelling opportunities that can deliver real value for manufacturers.
Increase manufacturing operational efficiency
Improving efficiency across the business helps a manufacturing company control costs, increase productivity, and boost margins. Automated production lines are already standard practice for many, but manufacturing big data can exponentially improve line speed and quality. For example, ML-driven analysis of automated test results such as photographs, X-rays, temperature measurements and other outputs is inherently superior to manual processes for spotting anomalies in product quality. It lets manufacturers minimize human error and identify the parameters most likely to affect quality, while exponentially increasing the number of products they can inspect and ship in a given timeframe.
Manufacturing big data also increases transparency into the entire supply chain—for example, by using sensor and RFID data to track the location of tools, parts, and inventory in real time, reducing interruptions and delays. Companies can also increase supply chain transparency by analyzing individual processes and their interdependencies for opportunities to optimize everything from demand forecasting and inventory management to price optimization.
For a real-world example of manufacturing big data analytics in action, let’s look to the skies. Sensors incorporated into Rolls-Royce aircraft engines gather 70 million data points a year for real-time analysis by AI, ML, and sophisticated analytic tools. Rolls-Royce engineers use this data to manage and service the engines remotely, identifying and correcting potential performance issues before they become catastrophic. The company also uses advanced analytics to simulate engine designs and production processes for rapid testing and iteration.
Identify hidden risks in products and processes
Analyzing data about equipment wear and past failures allows a manufacturer to predict the life cycle of its equipment and set up appropriate predictive maintenance schedules that are time-based (based on a set time interval, such as every three weeks) or usage-based (based on how a piece of equipment has been used, such as every 10 production runs). That in turn helps to detect anomalies, minimizes downtime and waste, and helps the company make an optimal recovery plan in the event of an unexpected failure. In fact, a report from PWC and Mainnovation notes that widespread adoption of predictive maintenance could:
- Reduce costs by 12%
- Improve uptime by 9%
- Cut safety, health, environment, and quality risks by 14%
- Extend equipment lifetime by 20%
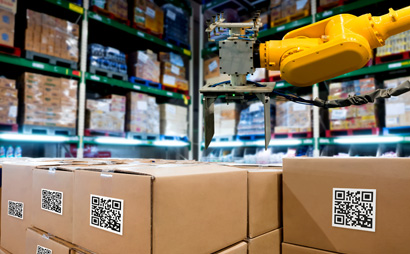
Machine learning also helps manufacturers analyze the yield and throughputs of each piece of equipment so they can identify areas for improvement at the individual machine level, in the associated workflows, and across the overall supply chain. This helps minimize overproduction and idle time while supporting better management of inventory and logistics. For example, manufacturers can use big-data-driven ML analysis to determine when to produce certain orders to optimize delivery or reduce the need for storage.
Combining AI with trusted big data and analytics offers manufacturers another risk-reducing opportunity: automating processes so they can self-optimize without human intervention. One cement company cited by McKinsey installed an AI-driven process optimizer to monitor and adjust the performance of its vertical mill and kiln in real time. After just eight months, the project allowed the company to run its production operations in autopilot mode, improving its feed rate per hour by 11.6% over manual mode and 9.6% over advanced process controls without AI.
Optimize pricing and configuration with AI and ML
AI-driven analysis of manufacturing big data enables companies to aggregate and analyze both their own and competitors' pricing and cost data to produce continually optimized price variants. For manufacturers that focus on build-to-order products, ML can also ensure the accuracy of their customized configurations and streamline the configure-price-quote (CPQ) workflow.
Railway control equipment from Siemens, for example, comes in trillions—1090 to be precise—of possible combinations. Applying AI and ML to data from thousands of past projects allows Siemens to determine which configuration best meets a customer's specific needs and from where it should be manufactured and delivered for optimal profit.
Evolve manufacturing analytics with data engineering: Accelerate digital transformation
The most powerful use of manufacturing big data, of course, is not in optimizing separate processes but in combining them. If your predictive maintenance report tells you when a part is likely to fail, you can schedule the replacement downtime in advance and choose a time that will have the least impact on your production and maintenance workloads. Looking at that in combination with your supply chain information will tell you when to order the new part—soon enough to be sure it's on hand when you need it, but not so early that you have to store it in your warehouse for weeks. And if that data dovetails with your sales and distribution systems, you can manage your replacement timeline to ensure you aren't doing a repair just when you're supposed to be completing and shipping a major order.
Data engineering is designed to make it easier to do all of this: combine your data resources and make trusted data accessible to the people and systems that use it. Big data engineering solutions help you ingest, prepare, and process massive amounts of high-volume data for data-hungry AI and ML systems. For manufacturers dealing with always-on streams of sensor and device data—as well as customer data, transaction data, and supplier data—building efficient data pipelines is critical to realizing the full value of AI in 2020 and beyond.
Manufacturing big data downloads and resources
- Blog: The Rise of Big Data Engineering
- White paper: Pursue a higher perfect order index score with more timely, accurate metrics about your supply chain
- Learn more about big data characteristics and how to address no-limits big data