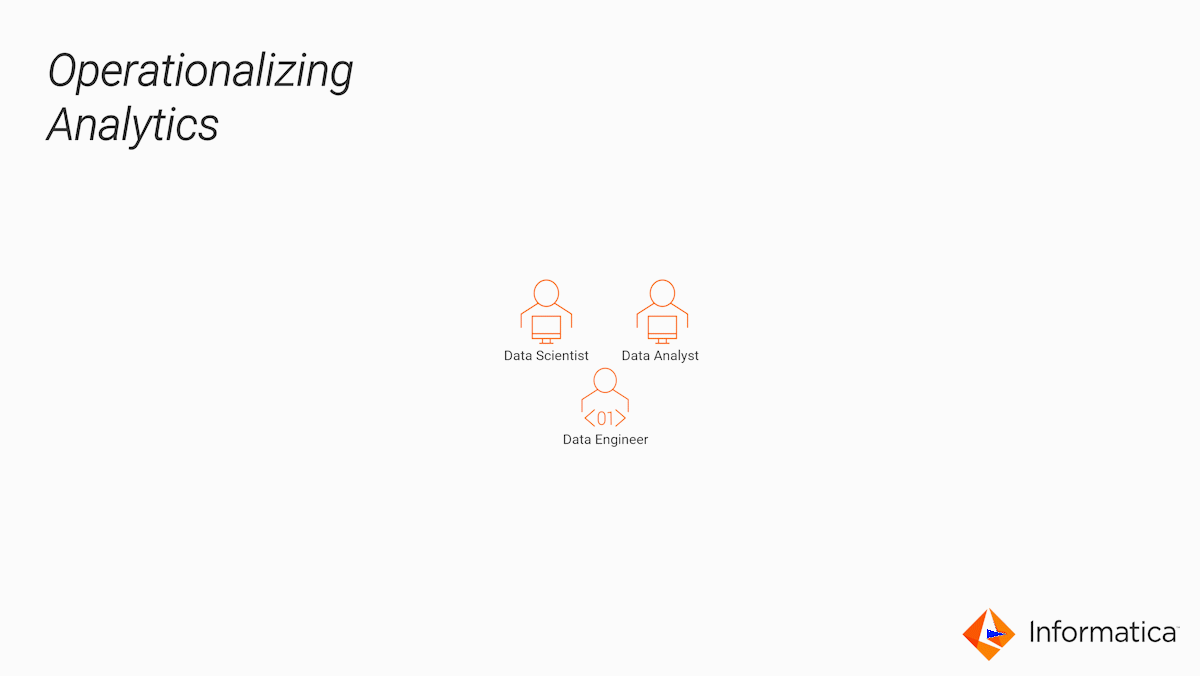
What is data analytics?
Data analytics is the strategy and process of organizing and analyzing vast volumes of data to drive more informed enterprise decision-making. Enterprise analytics tools import and store data in a cloud data lake, then transform and process it at scale, and finally add data quality rules and lineage—a data pipeline process known as data engineering. Data scientists, data analysts, and other users can then leverage this clean, trusted, high-quality data by applying predictive modeling, machine learning, and other advanced analytic tools to drive trusted decisions.
Data analytics is generally cloud-based, which makes it faster, more affordable, and easier to maintain than legacy analytics processes. It generally goes beyond structured data to tap into semi-structured and unstructured data, including mobile, social, IoT, and clickstream data. Adopting analytics for data thus enables you to identify correlations, trends, customer preferences, and other strategically relevant patterns in your data that would otherwise remain hidden. (Learn more about data use cases here).
Five immediate benefits of data analytics
Data analytics tend to fall into four categories, each answering a different business question:
- Descriptive: What happened in the past and when?
- Diagnostic: Where, how, and why did it happen?
- Predictive: What is likely to happen next?
- Prescriptive: What is the best thing to do now?
When you can answer any or all of those questions confidently and accurately, you can deliver measurable benefits:
- New revenue opportunities: Enterprise data analytics let you harness your data to identify underserved markets and untapped opportunities so you can develop new products and services that fill those unmet needs.
- More effective marketing: Data analytics help you evaluate customer purchasing patterns and satisfaction levels at scale so you can improve conversion rates with precisely targeted and highly personalized marketing campaigns.
- Better customer service: Because data analytics tools can deliver relevant information about customers in real time (from past interactions to social media sentiment), your front-office employees and automated systems can respond appropriately to enhance customer experience and increase retention and loyalty.
- Improved operational efficiency: Enterprise analytics tools can help organizations predict and identify opportunities to streamline operational processes by boosting productivity, optimizing pricing, minimizing risk, preventing fraud, using available resources more effectively, and more.
- Competitive advantage: The point of data analytics is not simply to analyze more data in less time, but to generate immediately actionable insights so you can create and implement your strategy faster than your competition. Whether that means refining the supply chain, spotting areas for cost savings, forecasting purchasing trends, or improving product recommendations and personalized offers, being data-driven is what keeps you out in front of your rivals.
Five requirements for effective data analytics
Realizing the benefits of data analytics takes more than just a few adjustments to traditional data management systems. It calls for an end-to-end, modern data management framework that takes advantage of cloud flexibility, applies intelligent automation to core tasks, and incorporates data protection, quality, and governance from the very start. Prioritizing these requirements ensures that your data analytics systems always work with the best possible data.
1. Build in the cloud
The cloud is inherently ready for data: cloud processing power and storage both scale elastically to accommodate large volumes of data, of any type, at any speed. Only data engineering makes it feasible to use cloud data lakes for storage so you can integrate, synchronize, and relate all enterprise data, applications, and processes.
2. Operationalize your data pipelines
Data engineering integration with an easy-to-use interface is faster than hand-coding for automating processes, facilitating collaboration, and building data pipelines quickly. The faster you can process it into on-premises systems, cloud repositories, and messaging hubs like Apache Kafka, the sooner you can make it available for real-time processing and timely, accurate reports.
3. Discover the right data
Analytics are only as good as the data being analyzed. A metadata-based data catalog that incorporates machine learning to first discover all sources of data, and then keep your data correct, complete, and relevant by classifying and organizing data assets across any environment to maximize data value and reuse while creating a metadata system of record.
4. Ingest the right data
Once you know what data you have, you must be able to ingest it seamlessly in massive amounts from multiple sources. That also means ingesting a wide variety of data, from files and databases to IoT and streaming. Make sure you can process ingested data at scale and load it into your cloud data lake.
5. Ensure that trusted data is available for insights
Data must be reliably high quality, complete, and consistent to be trusted. Look for a scalable, role-based data quality solution that applies data quality rules right out of the box—and can even recommend the right data quality rules to apply—to ensure that all the data in your data lake is fit for use and ready to deliver deep insights that drive solid business decisions.
Customer success stories: Data analytics in action
AXA XL
As the insurance industry becomes increasingly commoditized, AXA XL seeks to differentiate itself with a data-driven strategy, reinventing and redesigning the company through a multi-phase digital transformation. However, due to M&A-fueled growth, AXA XL’s data repositories existed in silos, with limited its ability to fully use the data to gain insights into the performance of customers, brokers, and products. Using data to drive strategy was complex, time consuming, and expensive, with many hours spent collecting, cleansing, and reconciling data across the firm.
AXA XL completely redesigned and consolidated its data architecture with a cloud-based data warehouse, advanced analytics platform, and business intelligence tools across the insurance value chain. Informatica’s suite of integrated products and unified support enables AXA XL to integrate, govern, and cleanse data from various cloud and on-premises sources, as well as make data easy to find and prepare for analysis.
With the ability to quickly perform robust analyses, actuaries can supply business leaders with actionable information, helping them make the right decisions about risk selection and the introduction of new products. The platform is already increasing stakeholder value by enabling complex activities such as cross-selling and upselling of insurance policies through brokers and managing general underwriters.
MD Anderson Cancer Center
MD Anderson Cancer Center launched the Moon Shots Program to target six forms of cancer with large multi-disciplinary clinical and research teams. The goal: to make inroads against the disease while improving survival and quality of life for patients.
The organization also works to accelerate the implementation of personalized cancer medicine by rapidly disseminating changes and improvements in clinical practice to improve patient outcomes globally. By harnessing data, the organization can reduce clinical trial cohort selection time and speed time to discovery of evidence. It needed to create a single source of hugely disparate longitudinal patient data, operational data and genomic data to power insight discovery, clinical decision support, and business analytics.
Informatica helped MD Anderson build a data analytics platform that securely houses clinical and genomics data in one centralized location. By harnessing data, the organization can reduce clinical trial cohort selection time and speed time to discovery of evidence.
Getting started with data analytics
Data analytics tools have enormous potential to transform your organization by accelerating innovation, increasing operational efficiency, enhancing customer service, and lowering costs. To optimize their value, though, you need to ensure that the data they analyze is clean, trusted, high-quality, and relevant. Informatica’s data engineering solutions are designed to help you find, ingest, process, govern, and prepare data so you can turn it into faster, more accurate business insights.
Learn more about data analytics and data engineering with these resources:
E-book: Next-Gen iPaaS For Dummies, Informatica Special Edition
On-Demand Virtual Summit: Data for AI and Analytics
Webinar: Turn Real-Time Customer Activity into Real-Time Revenue Through Data
E-book: Cloud Analytics for Dummies
Webinar: 7 Ways You Can Optimize Your Data Quality Practices for