What Is Data Lineage?
Data lineage essentially helps to determine the data provenance for your organization. It can provide an ongoing and continuously updated record of where a data asset originates, how it moves through the organization, how it gets transformed, where it’s stored, who accesses it and other critical metadata.
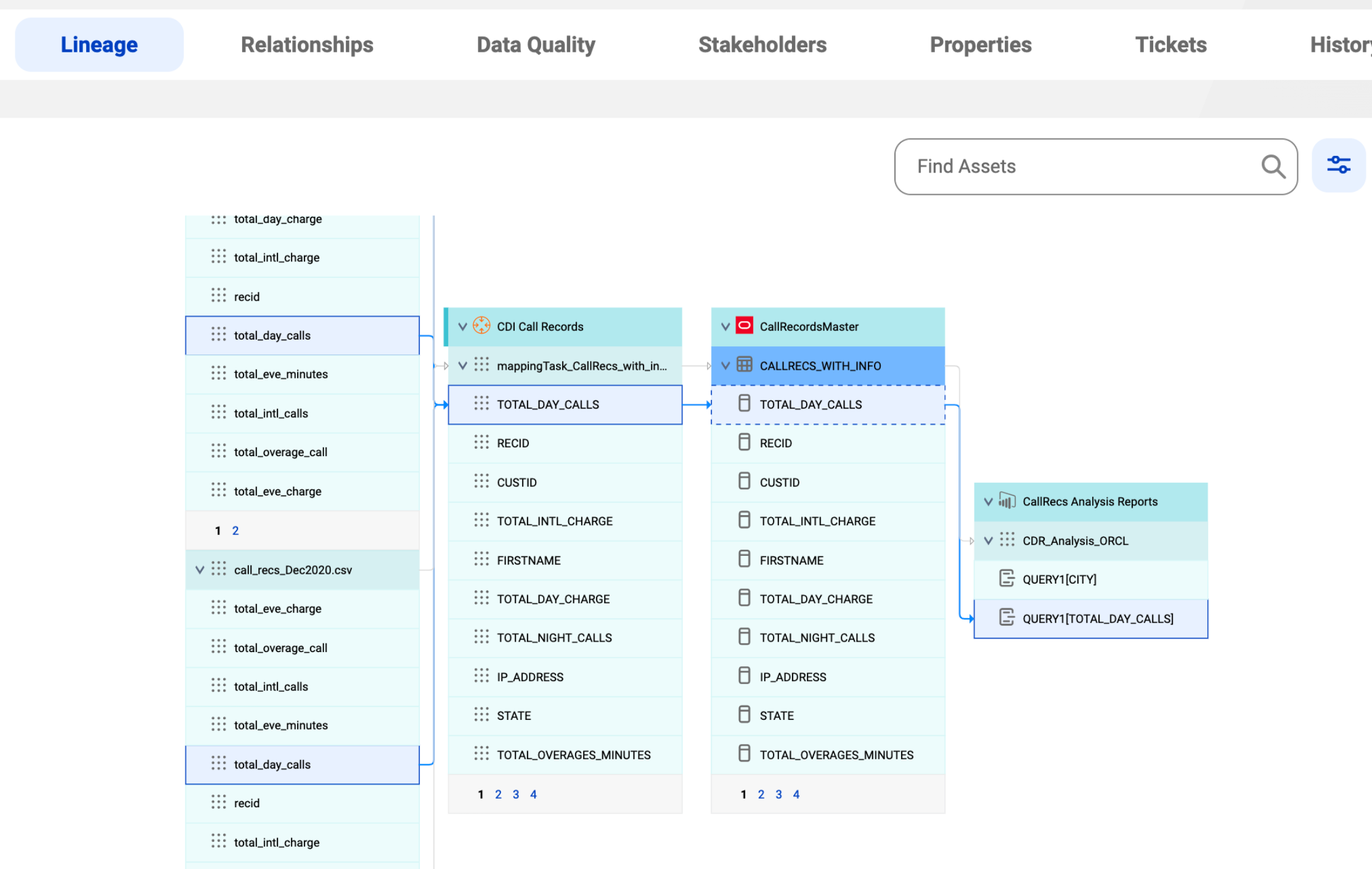
Example of data lineage as seen on the Informatica platform.
Data lineage answers the question, “Where is this data coming from, and where is it going?” It is a visual representation of data flow that helps track data from its origin to its destination. It explains the different processes involved in the data flow and their dependencies. Metadata management is critical to capturing enterprise data flow and presenting data lineage across cloud and on-premises environments.
What Questions Does Data Lineage Answer?
Data lineage clarifies how data flows across the organization. This includes the availability, ownership, sensitivity and quality of data. It helps ensure that you can generate confident answers to questions about your data:
- What data in my enterprise needs to be governed for compliance with local and industry regulations?
- What data sources have the personal information needed to develop new customer experience initiatives? And how is this data distributed across the organization?
- What data is appropriate to migrate to the cloud and how will this affect users?
- Where do we have data flowing into locations that violate data governance policies?
- How does data quality change across multiple lineage hops?
- How can data scientists improve confidence and trust in the data needed for advanced analytics?
Why Is Data Lineage Important?
Data lineage is essential for regulatory compliance, data quality, data privacy and security. It’s also vital for data analytics and data science initiatives. The ability to map and verify how data has been accessed and modified is critical for data transparency. It helps in generating a detailed record of where specific data originated. It also shows how data has been changed, impacted and used. Data lineage also makes it easier to respond to audit and reporting inquiries for regulatory compliance. Additionally, it helps increase security posture by enabling organizations to track and identify potential risks in data flows.
Data lineage helps organizations take a proactive approach to identifying and fixing gaps in data required for business applications. This is particularly useful for data analytics and customer experience programs. Collecting sensitive data exposes organizations to regulatory scrutiny and business abuses. Data lineage shows how sensitive data and other business-critical data flows throughout your organization. This way you can ensure that you have proper policy alignment to the controls in place.
For IT operations, data lineage helps visualize the impact of data changes on downstream analytics and applications. It also helps to understand the risk of changes to business processes. And it enables you to take a more proactive approach to change management. It also drives operational efficiency by cutting down time-consuming manual processes and enables cost reduction by eliminating duplicate data and data silos.
In addition, data lineage helps achieve successful cloud data migrations and modernization initiatives that drive transformation. Data lineage can help visualize how different data objects and data flows are related and connected with data graphs and graphic overlays. This deeper understanding makes it easier for data architects to predict how moving or changing data will affect the data itself. Predicting the impact on the downstream processes and applications that depend on it and validating the changes also becomes easier.
Data Lineage Best Practices
Here are a few things to consider when planning and implementing your data lineage.
Automate data lineage extraction
Many organizations today rely on manually capturing lineage in Microsoft Excel files and similar static tools. That practice is not suited for the dynamic and agile world we live in where data is always changing.
Include the source of metadata in data lineage
ETL software, BI tools, relational database management systems, modeling tools, enterprise applications and custom applications all create their own data about your data. This metadata is key to understanding where your data has been and how it has been used, from source to destination.
Involve owners of metadata sources in verifying data lineage
Communicate with the owners of the tools and applications that create metadata about your data. They know better than anyone else how timely, accurate and relevant the metadata is.
Plan progressive extraction of the metadata and data lineage
Trace the path data takes through your systems. Then, extract the metadata with data lineage from each of those systems in order. This makes it easier to map out the connections, relationships and dependencies among systems and within the data.
Validate end-to-end lineage progressively
Start by validating high-level connections between systems. Then, drill down into the connected data set, followed by data elements. Finally, validate the transformation level documentation.
Use an enterprise-class data catalog
For granular, end-to-end lineage across cloud and on-premises, use an intelligent, automated, enterprise-class data catalog sources to stitch together lineage from all your enterprise sources automatically. This includes the ability to extract and infer lineage from the metadata.
4 Data Lineage Techniques to Start Using Now
Ensure you have a breadth of metadata connectivity
For end-to-end data lineage, you need to be able to scan all your data sources across multi-cloud and on-premises enterprise environments. This ranges from legacy and mainframe systems to custom-coded enterprise applications and even AI/ML code. AI-powered data discovery capabilities can streamline the process of identifying connected systems. This can include using metadata from ETL software and describing lineage from custom applications that don’t allow direct access to metadata.
Take advantage of AI and machine learning
AI and machine learning (ML) capabilities can infer data lineage when it’s impracticable or impossible to do so by other means. Similar data has a similar lineage. But sometimes, there is no direct way to extract data lineage. For example, it may be the case that data is moved manually through FTP or by using code. In this case, with predictive data intelligence, AI-powered data similarity discovery enables you to infer data lineage by finding like datasets across sources. AI and ML capabilities also enable data relationship discovery. This is essential for impact analysis.
AI-powered data lineage capabilities can help you understand more than data flow relationships. It also brings insights into “control” relationships, such as joins and logical-to-physical models. For example, deleting a column that is used in a join can impact a report that depends on that join. An AI-powered solution that infers joins can help provide end-to-end data lineage. This enables a more complete impact analysis, even when these relationships are not documented.
Extract deep metadata and lineage from complex data sources
It’s a challenge to gain end-to-end visibility into data lineage across a complex enterprise data landscape. One that typically includes hundreds of data sources. This could be from on-premises databases, data warehouses and data lakes, and mainframe systems. Or it could come from SaaS applications and multi-cloud environments. For comprehensive data lineage, you should use an AI-powered solution. One that automatically extracts the most granular metadata from a wide array of complex enterprise systems. This includes ETL software, SQL scripts, programming languages, code from stored procedures, code from AI/ML models and applications that are considered “black boxes.”
Provide different capabilities to different users
Giving your business users and technical users the right type and level of detail about their data is vital. It helps them understand and trust it with greater confidence. Having access increases their productivity and helps them manage data. And it links views of data with underlying logical and detailed information. This improves collaboration and lessens the burden on your data engineers.
Data Lineage Customer Success Stories
Insurance firm AIA Singapore needed to provide users across the enterprise with a single, clear understanding of customer information and other business data. Data lineage helped them discover and understand data in context. It also enabled them to keep quality assurances high to optimize sales, drive data-driven decision making and control costs.
An industry-leading auto manufacturer implemented a data catalog to track data lineage. This provided greater flexibility and agility in reacting to market disruptions and opportunities. As a result, it’s easier for product and marketing managers to find relevant data on market trends. They can also trust the results of their self-service reporting — thus reaching actionable insights 70% faster.
Data Lineage: Catalyst for Digital Transformation
Informatica’s AI-powered data lineage solution, part of the Intelligent Data Management Cloud, includes a data catalog with data discovery capabilities. This helps ensure you capture all the relevant metadata about all your data from all your data sources. It also provides detailed, end-to-end data lineage across cloud and on-premises environments. Learn more about why data lineage is critical and how CDOs/CDAOs use it to help drive growth and transformation with our eBook, “ AI-Powered Data Lineage: The New Business Imperative.”
Additional Data Lineage Resources
Register for our webinar series: Data Lineage in the Cloud.
Discover how AI-powered data lineage is key to greater data intelligence and trust.