What Is Metadata Management?
Metadata management is critical to building a data-driven business. It consists of best-practice processes and technologies that allow you to manage this data about your data. Metadata management helps provide you with insights for more effective data management. And it allows users of all kinds — business and technical — to search for, understand and access the data they need to do their jobs.
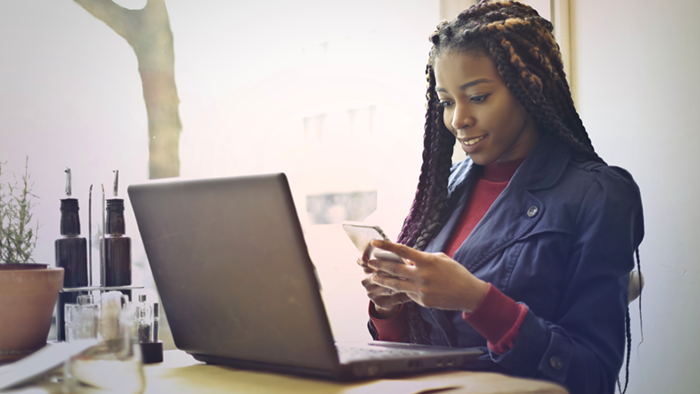
So, what is metadata?
Metadata is data about data. It provides the context you need to use your data effectively and unleash its full value. Specifically, metadata helps you discover data. It helps you understand the relationships between different pieces of data. Metadata lets you track how data is used, and assess the benefits and risks associated with that use.
Data continues to grow at an explosive rate. It has also become more distributed and now fuels many mission-critical processes. And that is why metadata management now plays a central, strategic role in driving digital transformation.
Why Is Metadata Management Important?
The right metadata management tools provide full context to help you understand your data. They help improve data quality and relevance. This allows you to unleash your data’s full business value. As data continues to grow and become more distributed, it’s essential to use metadata to:
- Discover data
- Understand data relationships
- Track how data is used
- Assess the value and risks associated with data usage
These are mission-critical processes. As such, metadata management plays a central, strategic role in supporting the CDO’s goals and driving digital transformation.
A metadata management solution becomes even more valuable when it is supported by AI & machine learning. These intelligent solutions are considered “active” and can be augmented with human knowledge and integrated with data analytics programs and other applications to generate new insights from your data. This makes broader data management processes, applications and systems more intelligent and dynamic.
Managing the Breadth of Data
Metadata management must be able to handle the breadth of data across the enterprise. There are two dimensions to this breadth. One deals with the ability to collect metadata from sources across the enterprise, both on-premises and cloud. The other reflects the four different types of metadata that must be managed:
- Technical metadata, including database schemas, mappings and code, transformations and quality checks
- Business metadata, including glossary terms, governance processes, and application and business context metadata
- Operational and infrastructure metadata, including run-time stats, time stamps, volume metrics, log information and system and location
- Usage metadata, including that for user rating, comment and access-pattern
As mentioned in the previous section, active metadata is metadata that is overlaid with AI and machine learning. Instead of helping users simply analyze metadata, active metadata management and predictive data intelligence recommend or trigger action. For example, metadata can flag missing or incorrect data, then automatically correct and enrich the metadata. This helps improve the quality of analytics, avoids costly mistakes and enhances decision-making.
How Does Metadata Management Work?
Metadata in the four categories described above becomes the basis for a common metadata foundation. Intelligent metadata management tools use a rich set of capabilities to create this shared foundation:
- Collect
Scan metadata from enterprise data systems across the cloud and on-premises, such as data lakes and data warehouses. This includes databases and filesystems, data integration tools and processes that help fuel your data analytics and data science tools with a high level of trustworthiness toward data fitness.
- Curate
Document the business view of data with glossary terms, concepts, relationships and processes. Augment the collected metadata with this business context. Gather user input in the form of ratings, reviews and certifications. This helps to assess the usefulness of data assets to other users.
Apply intelligence to derive relationships not obvious in the collected metadata. This includes data provenance and data lineage, data similarity and ranking the most useful data sets for different types of users and purposes.
Seven Best Practices for Metadata Management
These best practices for metadata management will help you scale intelligent data management for the modern multi-cloud and hybrid data environment:
- Build a unified metadata foundation: Create a common metadata foundation to deliver insight and intelligence across all your data management processes.
- Tap into four major categories of metadata: Harness the full value of your data with metadata-driven intelligence across these four types of metadata: technical, business, operational/infrastructure and usage/social.
- Apply AI/ML to activate your metadata: Make all cloud data management processes intelligent and dynamic by applying AI/ML on top of unified metadata management.
- Leverage an AI-powered data catalog to make metadata visible and consumable for all data consumers: Capture enterprise metadata and add valuable business context to it so data has value throughout the organization. An AI-powered data catalog scans, catalogs and indexes metadata, helping consumers across your organization easily discover, understand and trust relevant data.
- Ensure breadth, depth and scale of metadata management: Meet modern data landscape demands with metadata management that has breadth of metadata connectivity across multi-cloud, on-premises, applications, ETL tools and BI tools; depth to extract deep metadata and understand detailed data lineage (e.g., by parsing code in databases); and massive scale to support hundreds of millions of metadata assets.
- Enable AI/ML model governance: Operationalize AI/ML models by capturing and managing metadata for the models. This enables governance of AI/ML models to measure performance against business KPIs and avoid algorithmic bias.
- Develop a metadata stewardship program: Include metadata stewards on your data governance team, who can take the high-level metadata management policies and implement them in practice. This is often a key indicator of a successful metadata management strategy.
Customer Success Story
Global insurance and reinsurance company AXA XL has more than 100 offices across six continents. They wanted to standardize on a single data management solution with integrated products to manage all of their metadata as they deployed their cloud infrastructure, which fed a Microsoft Azure-based data lake.
With Informatica, they were able to make data actionable and easy to find. This resulted in more profitable growth through cross-selling and upselling of insurance policies. They were also able to democratize data discovery and preparation. This delivered faster and deeper insights to data scientists, analysts and actuaries. And that resulted in a quicker time to market for new types of policies and coverages.
Evolve Your Approach to Metadata Management
For any business serious about its data, metadata management is critical. You need a solution that lets you:
- Manage all types of metadata, across on-premises and multi-cloud environments
- Use intelligent active metadata management methods
- Follow best practices to get the most out of your investment in data
Additional Metadata Management Resources
Learn more about metadata and how to manage it by downloading our eBook, “Build the Data Foundation for Every Digital Transformation Priority.”
Read about trends in metadata management in this blog, “Metadata is Dead, Long Live Metadata.”
You’ll find more examples of how metadata management enables intelligent data management in this blog, Seven Metadata Management Best Practices Every Successful Data Leader Must Know.