What is ETL (extract transform load)?
Defining ETL
If you’re working with data warehouses and data integration, chances are you’re familiar with the acronym “ETL,” or “extract, transform and load.” It’s a three-step data integration process used by organizations to combine and synthesize raw data from multiple data sources into a data warehouse, data lake, data store, relational database or any other application. Data migrations and cloud data integrations are common use cases.
The ETL Process Explained
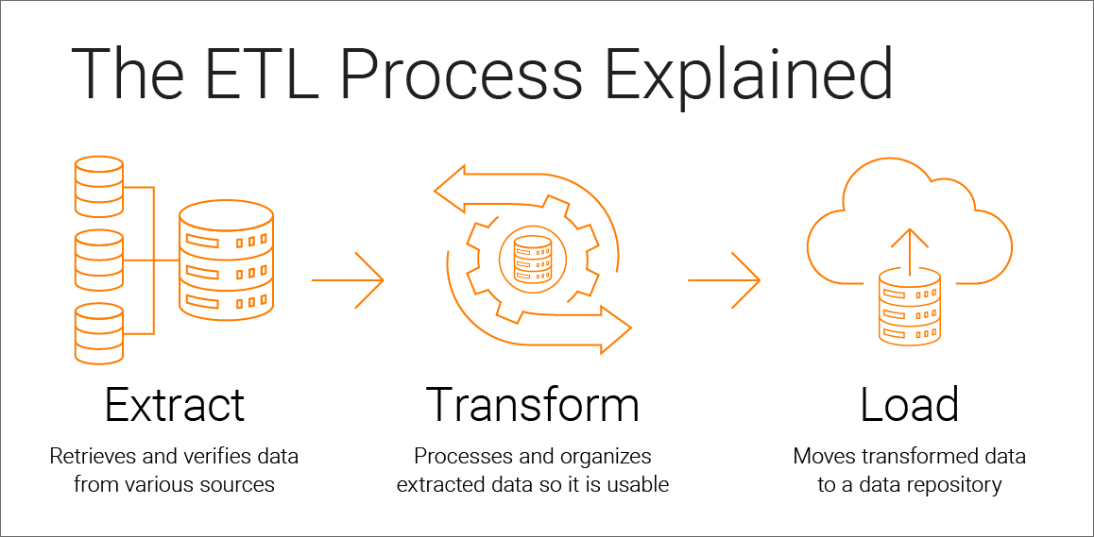
ETL moves data in three distinct steps from one or more sources to another destination. This could be a database, data warehouse, data store or data lake. Here’s a quick summary:
Extract
Extraction is the first phase of “extract, transform, load.” Data is collected from one or more data sources. It is then held in temporary storage, where the next two steps are executed.
During extraction, validation rules are applied. This tests whether data meets the requirements of its destination. Data that fails validation is rejected and doesn’t continue to the next step.
Transform
In the transformation phase, data is processed to make its values and structure conform consistently with its intended use case. The goal of transformation is to make all data fit within a uniform schema before it moves on to the last step.
Typical transformations include aggregators, data masking, expression, joiner, filter, lookup, rank, router, union, XML, Normalizer, H2R, R2H and web service. This helps to normalize, standardize and filter data. It also makes the data fit for consumption for analytics, business functions and other downstream activities.
Load
Finally, the load phase moves the transformed data into a permanent target system. This could be a target database, data warehouse, data store, data hub or data lake — on-premises or in the cloud. Once all the data has been loaded, the process is complete.
Many organizations regularly perform this process to keep their data warehouse updated.
Traditional ETL vs. Cloud ETL
Traditional ETL
Traditional or legacy ETL is designed for data located and managed completely on-premises by an experienced in-house IT team. Their job is to create and manage in-house data pipelines and databases.
As a process, it generally relies on batch processing sessions that allow data to be moved in scheduled batches. This ideally occurs when traffic on the network is reduced. Real-time analysis can be hard to achieve. To extract the necessary data analytics, IT teams often create complicated, labor-intensive customizations and exact quality control. Plus, traditional ETL systems can’t easily handle spikes in large data volumes. That often forces organizations to choose between detailed data or fast performance.
Cloud ETL
Cloud, or modern, ETL extracts both structured and unstructured data from any data source type. The data could be in on-premises or cloud data warehouses. It then consolidates and transforms that data. Next, it loads the data into a centralized location where it can be accessed on demand.
Cloud ETL is often used to make high-volume data readily available for analysts, engineers and decision makers across a variety of use cases.
ETL vs. ELT
Extract transform load and extract load transform are two different data integration processes. They use the same steps in a different order for different data management functions.
Both ELT and ETL extract raw data from different data sources. Examples include an enterprise resource planning (ERP) platform, social media platform, Internet of Things (IoT) data, spreadsheet and more. With ELT, raw data is then loaded directly into the target data warehouse, data lake, relational database or data store. This allows data transformation to happen as required. It also lets you load datasets from the source. With ETL, after the data is extracted, it is then defined and transformed to improve data quality and integrity. Then, it is subsequently loaded into a data repository where it can be used.
Which should you use? Consider ETL if you’re creating data repositories that are smaller, need to be retained for a longer period and don’t need to be updated very often. ELT is best if you’re dealing with high-volume datasets and big data management in real-time.
Check out our “What are the differences between ETL and ELT?” blog post for an in-depth overview.
ETL pipeline vs. data pipeline
The terms “ETL pipeline” and “data pipeline” are sometimes used interchangeably. However, there are fundamental differences between the two.
A data pipeline is used to describe any set of processes, tools or actions used to ingest data from a variety of different sources and move it to a target repository. This can trigger additional actions and process flows within interconnected source systems.
With an ETL pipeline, the transformed data is stored in a database or data warehouse. There, the data can then be used for business analytics and insights.
What are the different types of ETL pipelines?
ETL data pipelines are categorized based on their latency. The most common forms employ either batch processing or real-time processing.
Batch processing pipelines
Batch processing is used for traditional analytics and business intelligence use cases where data is periodically collected, transformed and moved to a cloud data warehouse.
Users can quickly deploy high-volume data from siloed sources into a cloud data lake or data warehouse. They can then schedule jobs for processing data with minimal human intervention. With ETL in batch processing, data is collected and stored during an event known as a “batch window.” Batches are used to more efficiently manage large amounts of data and repetitive tasks.
Real-time processing pipelines
Real-time data pipelines enable users to ingest structured and unstructured data from a range of streaming sources. These include IoT, connected devices, social media feeds, sensor data and mobile applications. A high-throughput messaging system ensures the data is captured accurately.
Data transformation is performed using a real-time processing engine like Spark streaming. This drives application features like real-time analytics, GPS location tracking, fraud detection, predictive maintenance, targeted marketing campaigns and proactive customer care.
The challenges of moving from ETL to ELT
The increased processing capabilities of cloud data warehouses and data lakes have shifted the way data is transformed. This shift has motivated many organizations to move from ETL to ELT. This isn’t always an easy change.
ETL mappings have grown robust enough to support complexity in data types, data sources, frequency and formats. Successfully converting these mappings into a format that supports ELT requires an enterprise data platform capable of processing data and supporting pushdown optimization without breaking the front end. What if the platform can’t generate the ecosystem or data warehouse-specific code necessary? Developers end up hand coding the queries to incorporate advanced transformations. This labor-intensive process is costly, complicated and frustrating. That’s why it’s important to select a platform with an easy-to-use interface that can handle replicating the same mappings and run in an ELT pattern.
Benefits of ETL
ETL tools work in concert with a data platform and can support many data management use cases. These include data quality, data governance, virtualization and metadata. Here are the top benefits:
Get deep historical context for your business
When used with an enterprise data warehouse (data at rest), ETL provides historical context for your business. It combines legacy data with data collected from new platforms and applications.
Simplify cloud data migration
Transfer your data to a cloud data lake or cloud data warehouse to increase data accessibility, application scalability and security. Businesses rely on cloud integration to improve operations now more than ever.
Deliver a single, consolidated view of your business
Ingest and synchronize data from sources such as on-premises databases or data warehouses, SaaS applications, IoT devices and streaming applications to a cloud data lake. This establishes a 360-degree view of your business.
Enable business intelligence from any data at any latency
Businesses today need to analyze a range of data types. This includes structured, semi-structured and unstructured data. And data from multiple sources, such as batch, real-time and streaming.
ETL tools make it easier to derive actionable insights from your data. As a result, you can identify new business opportunities and guide improved decision-making.
Deliver clean, trustworthy data for decision-making
Use ETL tools to transform data while maintaining data lineage and traceability throughout the data lifecycle. This gives all data practitioners, ranging from data scientists to data analysts to line-of-business users, access to reliable data.
Artificial intelligence (AI) and machine learning (ML) in ETL
AI and ML-based ETL automates critical data practices, ensuring the data you receive for analysis meets the quality standard required to deliver trusted insights for decision-making. It can be paired with additional data quality tools to guarantee data outputs meet your unique specifications.
ETL and data democratization
Technical users aren’t the only ones who need ETL. Business users also need to easily access data and integrate it with their systems, services and applications. Infusing AI in the ETL process at design time and run time makes this easy to achieve. AI- and ML-enabled ETL tools can learn from historical data. The tools can then suggest the best reusable components for the business users’ scenario. This could include data mappings, mapplets, transformations, patterns, configurations and more for the business users’ scenario. The result? Increased team productivity. Plus, automation makes policy compliance easier since there’s less human intervention.
Automate ETL pipelines
AI-based ETL tools enable time-saving automation for onerous and recurring data engineering tasks. They allow you to gain data management effectiveness and to accelerate data delivery. And you can automatically ingest, process, integrate, enrich, prepare, map, define and catalog data for your data warehousing.
Operationalize AI and ML models with ETL
Cloud ETL tools let you efficiently handle the large data volumes required by data pipelines used in AI and ML. With the right tool, you can drag and drop ML transformations into your data mappings. This makes data science workloads more robust, efficient and easier to maintain. AI-powered ETL tools also let you easily adopt continuous integration/continuous delivery (CI/CD), DataOps and MLOps to automate your data pipeline.
Replicate your database with change data capture (CDC)
ETL is used to replicate and auto sync data from various source databases to a cloud data warehouse. The sources could include MySQL, PostgreSQL, Oracle and others. To save time and increase efficiency, use change data capture to automate the process to only update the datasets that have changed.
Greater business agility via ETL for data processing
Teams will move more quickly as this process reduces the effort needed to gather, prepare and consolidate data. AI-based ETL automation improves productivity. It lets data professionals access the data they need, where they need it, without needing to write code or scripts. This saves valuable time and resources.
Cloud ETL pricing: Things to consider
While ETL is implemented to share the data between a source and target, technically the data is copied, transformed and stored in a new place. This can affect pricing and resources. It all depends on whether you’re using on-premises ETL or cloud ETL.
Businesses using on-premises ETL have already paid for the resources. Their data is stored on location with an annual budget and capacity planning. But in the cloud, storage cost is different. It is recurring, usage-based and goes up every time you ingest and save the data somewhere else. This can strain your resources if you don’t have a plan.
When using cloud ETL, it’s important to evaluate cumulative storage, processing costs and data retention requirements. This ensures you’re using the right integration process for each use case. And it helps you optimize costs with the right cloud-based integration pattern and data retention policies.
ETL cases by industry
ETL is an essential data integration component across a variety of industries. It helps organizations increase operational efficiencies, improve customer loyalty, deliver omnichannel experiences and find new revenue streams or business models.
ETL in Healthcare
Healthcare organizations use ETL in their holistic approach to data management. By synthesizing disparate data organization-wide, healthcare firms are accelerating clinical and business processes. At the same time, they’re improving member, patient and provider experiences.
ETL in the Public Sector
Public sector organizations operate on strict budgets. As such, they use ETL to surface the insights they need to maximize their efforts. Greater efficiency is vital to providing services with limited available resources. Data integration makes it possible for governmental departments to make the best use of both data and funding.
ETL in Manufacturing
Manufacturing leaders transform their data for many reasons. It can help them optimize operational efficiency. It also helps ensure supply chain transparency, resiliency and responsiveness. And it improves omnichannel experiences while ensuring regulatory compliance.
ETL in Financial Services
Financial institutions use ETL to access data that is transparent, holistic and protected to grow revenue. It helps deliver personalized customer experiences. It also enables them to detect and prevent fraudulent activity. They can also realize fast value from mergers and acquisitions while complying with new and existing regulations. This helps them understand who their customers are and how to deliver services that fit their specific needs.
Informatica and cloud ETL for data integration
Informatica offers industry-leading data integration tools and solutions to give you the most comprehensive, codeless, AI-powered cloud-native data integration. Create your data pipelines across a multi-cloud environment consisting of Amazon Web Services, Microsoft Azure, Google Cloud, Snowflake, Databricks and more. Ingest, enrich, transform, prepare, scale and share any data at any volume, velocity and latency for your data integration or data science initiatives. It can help you quickly develop and operationalize end-to-end data pipelines and modernize legacy applications for AI.
Get started with ETL
Sign up for the free Informatica Cloud Data Integration trial to experience first-hand how broad out-of-the-box connectivity, codeless, prebuilt advanced transformations and orchestrations can help accelerate your data pipelines. Informatica tools are easy to integrate and use whether you need single-cloud, multi-cloud or on-premises data integration.