Clarify Yourself, AI
Algorithmic decision making has become prevalent in systems such as online search, social media, productivity software, and autonomous vehicles. While these intelligent systems powered by AI offer tremendous benefits, the algorithmic decision making behind them often feels like a mystery.
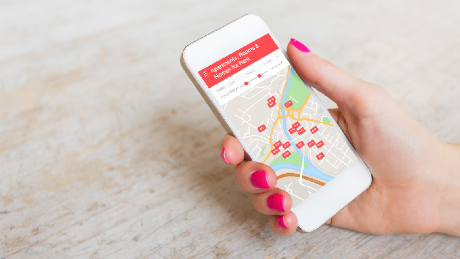
Without giving much thought to it, I used to blindly follow my friends’ activities on Facebook. I expected all my Facebook friends’ posts to appear in my news feed because I’m connected to them. When things had gone quiet, I had assumed that my friends and family were either not posting, or that they were actively hiding posts from me. That is, until I learned that the content that I see on Facebook is algorithmically curated.
After realizing that Facebook filters and ranks all available posts from my Facebook friends, I started to wonder how Facebook selects and controls the ordering and presentation of posts. Moreover, I began to speculate on how Facebook tracks my actions, so they could use them as signals to determine what content to display on my news feed.
Rather than attributing my lack of awareness about the Facebook’s content-curation algorithm to my own ignorance, I see this as an example of AI-driven products lacking transparency. User uncertainty and lack of knowledge about algorithmic decision making may cause anxiety (e.g., suspecting Facebook deliberately conceals posts). Worse, algorithmic avoidance could develop when users have a lower level of confidence in algorithmic than in human recommendations, even if the algorithmic recommendations are more accurate and insightful. This is why AI-enabled products must clarify the reasoning behind their predictions, recommendations, and other system decisions, so that we, as users, can understand, appropriately trust, and responsibly leverage the outputs from decision-making applications.
UX for AI design principle: Clarify
To enhance the productivity of all users with the power of CLAIRE, Informatica's intelligence engine, our user experience (UX) design approach considers the principle of Clarify when we design for AI-driven experiences. We understand and respect that users need and desire explanations, because the rationale behind algorithmically generated outcomes can be crucial to helping them make informed decisions and assessing the right actions to take.
To that end, when we clarify AI, we consider the following:
- Use: “How do I use it?”
- Function: “What does it achieve?”
- Processing details: “How does it work?”, “What did it just do?”, “What would it have done if “x” were different?”
- Anticipation: “What will it do next?”
- Usability: “How much effort will this take?”
This way, users have clear understanding about how the system works, can make the right decisions, make the decisions faster, and most importantly, have greater confidence in the system.
What counts as a good explanation, however, depends on the knowledge that the users already have, as well as what additional information they need to understand. Thus, our approach is rooted in understanding the users’ existing knowledge, needs, and goals. This is our persona-based approach to design.
Below are a few examples of what we aspire to achieve with CLAIRE:
- Tailor to users’ explanation objectives. First, determine the reasons for clarification. Based on the objective, use explanations to justify an outcome, to control and prevent errors, or to discover information and gain knowledge.
- Adapt to users’ current context. The information that is of interest or relevant to users can depend on various factors. Provide more or fewer details according to certain criteria (such as their domain knowledge, tool familiarity, or the amount of effort they might be willing to provide at this point in time).
- Select the right explanatory content. Identify the most pertinent aspects that need to be part of the clarification (from a sometimes-infinite number of causes to be explained). Additionally, incorporate various styles or forms of explanations in order to optimize users’ understanding (e.g., item-based explanation or contrastive explanation "It says it is ‘X,’ but why is it not ‘Y’?"). Also, consider the perspective (positive or negative) in which a clarification will be presented. Sometimes a negative perspective—with appropriate details regarding why certain negative aspects could be accepted—can be more effective.
- Design the explanation presentation. Choose a presentation format (e.g., textual or visual) that enhances the interpretability of the system outcomes. In addition, determine when the explanation is to be displayed, (e.g., upon user request, always shown, or depending on the context).
- Pay attention to the tone of voice. Think of explanations as interactive conversations with users. Consider their characteristics and preferences, as well as their emotions and information needs for each clarification. Adjust the tone (e.g., level of formality, humor, respectfulness, enthusiasm, personalization) so that it fits each unique situation.
In other blog posts, we explore additional AI design principles that need to be considered when designing AI-enabled products, including the AI UX design principles of Trust , Simplify, Control, and Humanize.