Why you need a Cloud Data Management Maturity Model
Every organization today is investing in cloud and cloud analytics. You may be building a cloud data warehouse or data lake on AWS, Azure, GCP, Snowflake, or Databricks. Whether you are doing it for the first time or have done it many times on on-premises and are now looking to consolidate and modernize in the cloud, this new journey can raise many questions.
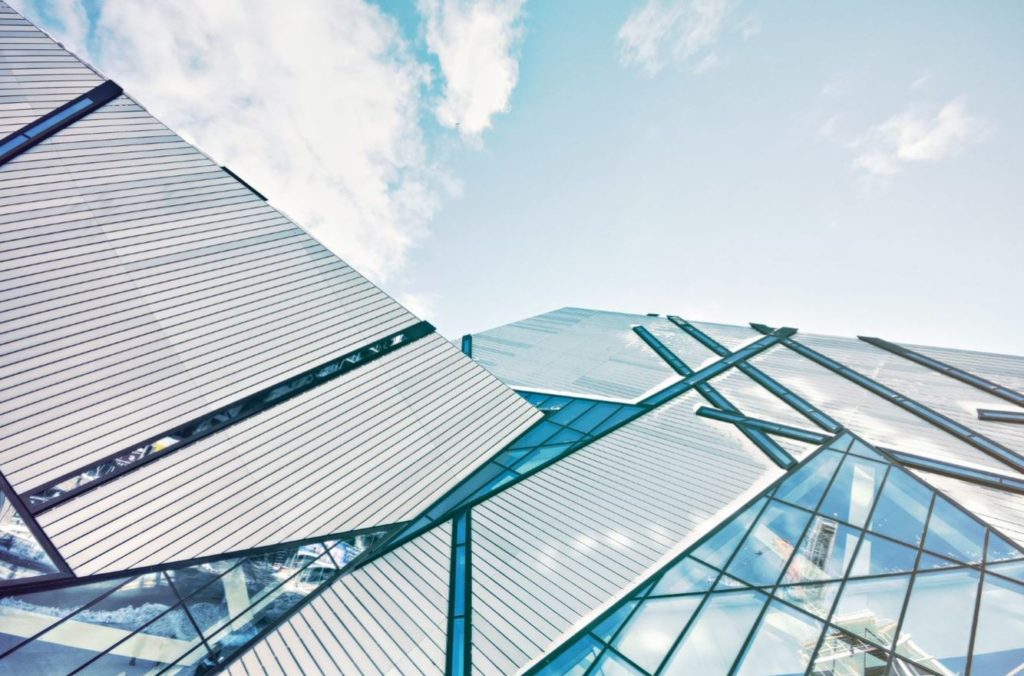
The most important question that comes to your mind is: How do I build these ETL/ELT data pipelines to manage and process data? Should I use Python, R, C++, C, or a tool-based solution? Those who have learned the hard way by building on-premises data warehouses may quickly come to a conclusion – we have been there, we have done that, and we know hand coding is costly, is a maintenance nightmare and does not easily provide data quality, governance, lineage, privacy, data ops and many more capabilities that a tool-based solution can offer. The second question you want to answer is: How can I align and consider industry best practices before starting that journey to cloud analytics?
The answer is similar to building a business, team, or you as an individual. You certainly need to understand how good you are doing and how well you are you are progressing. You want to set best practices and standards, review your performance against those standards, and course-correct, if you are deviating from those standards. A maturity model helps you to address these objectives.
So where can you find a maturity model for cloud analytics? If you are starting your cloud analytics journey, don’t miss the Maturity Model webinar on Cloud Data Management with Wayne Eckerson. You’ll learn about the Eckerson Maturity assessment and model, and you’ll come away with next steps that will help you make your cloud analytics journey successful.