Real-Time Data Drives Strategic Decisions
Decision making in business is about seeking a course of action from alternatives, and data powers the critical analysis to select the best course of action. Data has transformed the way businesses operate and take actions. Traditionally, organizations used data stored in their IT systems for making informed business decisions. Slowly, the decisions evolved to include using partner and external data to enrich the decision-making process. With the adoption of streaming analytics techniques and evolution of artificial intelligence (AI) and machine learning (ML), organizations are also ingesting the data in real-time so they can take immediate action on the data as it is being generated and as it is processed through the system. Gartner predicts that “By 2022, more than half of major new business systems will incorporate continuous intelligence that uses real-time context data to improve decisions.”1
In this blog, we will cover the following topics:
- Real-time data ingestion patterns
- Business use cases and initiatives with real-time data
- Real-time data ingestion technology enablers
- What to look for in a real-time data management solution
- How Informatica can help data engineers to adopt real-time data in an easy and consistent experience
The Need for Real-Time Data Ingestion Patterns
Real-time data is generated by a variety of sources and requires different data access and ingestion approaches depending on the sources and the business objectives. They include:
- Log data – events coming from application logs, clickstream, and weblogs
- Social media, HTTP, and REST data – continuous feeds from cloud and on-premises applications and social media platforms
- IoT data – continuous data from IoT sensors, devices, and gateways
- Change data capture (CDC) – change data from transactional systems
Gartner predicts that “By 2023, over 70% of organizations will use more than one data delivery style to support their data integration use cases, resulting in preference for tools than can support the combination of multiple data delivery styles (such as ETL and stream data integration).”2
Business Drivers and Use Cases
Following are some of the key business drivers where customers are leveraging streaming data for making strategic decisions:
- Enhance customer experience – with real-time offers, real time fraud detection, and real-time alerts
- Improve operational efficiency – with initiatives such as predictive maintenance and smart factory
- Real-time data driven decision making – with initiatives to reduce the latency of data consumption for real-time analytics
Real-time data use cases span across most verticals and segments. Some of the use cases are outlined below; note that this list is not an exhaustive set of use cases.
- In the manufacturing and oil and gas sector, real-time data is used in factory automation for operational efficiency and predictive maintenance for maximizing uptime and disaster tolerance.
- In the retail sector, with many retailers having both online and brick and mortar presence, retailers use streaming data for real-time offer management based on what customers buy and behavior analytics for how customers shop at the store.
- In the healthcare sector, hospitals leverage medical devices data for clinical research processes to enhance hospital experience and improve patient outcomes.
- In the financial services and insurance sector, banks and insurance companies leverage continuous feeds of transaction data to run fraud detection using machine learning models.
Real-Time Data Ingestion Technology Enablers
Many technologies contribute to the demand and increasing consumption of real-time data:
- Cheaper computing power – This has two variants: one at the edge where the data gets generated and the other where the data is processed. Computing power has increased leaps and bounds at the edge so that edge computing is now a reality – which helps customers to ingest clean data onto the lake, integrate the data, and reuse it for analytics. On the processing side, open source technologies such as Apache Spark have revolutionized the way compute scaling can be processed on commoditized hardware. These technologies are now helping solve complex use cases in real time, which was not possible before.
- Cheaper storage for large volumes of data – with the advent of cloud data lakes, it is now possible to store large amounts of streaming data at very low cost. Some cloud vendors don’t charge for storage, but only for compute, which helps spur the growth of data ingested into the lake.
- Rise of enterprise messaging systems – open source technologies such as Apache Kafka and cloud messaging systems like Amazon Kinesis and Azure Event Hubs have changed the way messaging systems are used. The messaging systems offer persistence, management, compute, and querying capabilities, which makes them ideal as the “hub” where data from various applications lands into Kafka and from which it can be consumed in various latencies – like batch, real-time, and interactive queries.
What to Look for in a Real-Time Data Management Solution
Your real-time data management solution should address the following requirements:
- Extend existing batch data management pipelines for real-time data: Almost all businesses have built data management pipelines for batch data sources like relational databases and files. The solution should extend the existing pipeline to include streaming data for investment protection and speed to market.
- Versatile connectivity: Real-time sources have a variety of standards, especially IoT data protocols including MQTT, OPC, AMQP, and others. It is also important to support structured, semi-structured, as well as unstructured data – so that the data can be easily parsed using machine learning techniques.
- Edge processing and enrichments: It is important to filter out erroneous records coming from the real-time sources (for example, negative temperature reading) before ingesting it onto the lake. Businesses also need to enrich the data with metadata (such as timestamp) so that more accurate analytics can be run on the data post ingestion.
- Address data/schema drift: Real-time data can change over time due to various events such as firmware upgrade, source system changes, or schema changes. This is referred to as data drift (or schema drift). It is important for the solution to automatically address the data drift without interruptions.
- Governance and lineage for real-time data: Data coming from real-time sources needs to be governed and managed in the same way as the batch data. Hence, end-to-end lineage for the data and overall governance of the data is very important.
Gartner predicts that “By 2022, over 75% of data integration tools will natively support stream data integration, resulting in fewer interoperability issues between different data integration tools and low-latency processing.”3
How Informatica Helps
Informatica’s approach to real-time data ingestion and management starts with collecting the raw data from the various sources and ingesting the data into the data lake or messaging hub. Informatica also offers data transformation and data enrichment capabilities to process the streaming data and make it available for operationalization and downstream analytics.
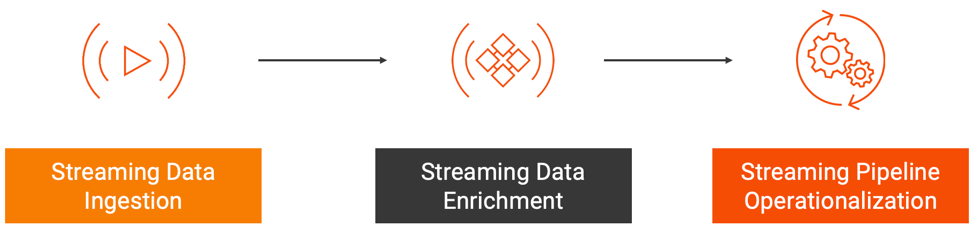
Informatica offers the Sense-Reason-Act framework for real-time data ingestion. The framework provides end to end data engineering capabilities to ingest real-time data, apply enrichments on the data in real-time or in batches, and operationalize the actions on the data in a single platform using a simple and unified user experience.
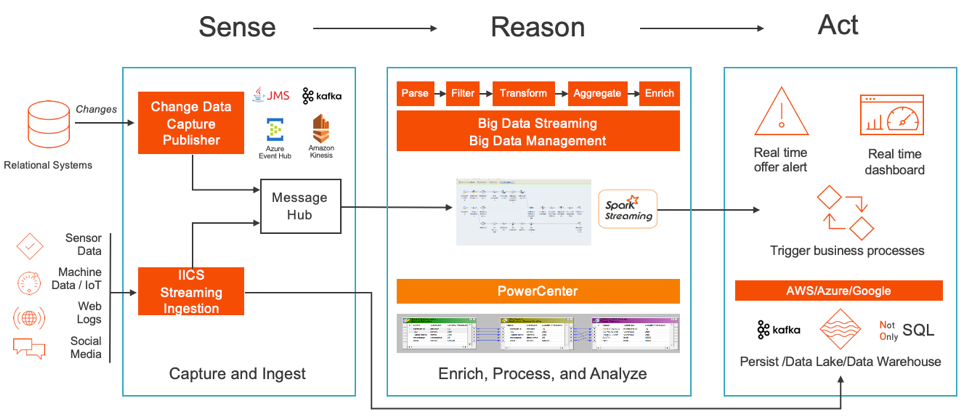
The need for real-time data in decision making is evolving rapidly within businesses due to increased benefits and competitive advantage. It is important for customers to understand how they can extend their data management platform to address the real-time data ingestion use cases. Informatica offers an end-to-end data engineering platform for addressing batch and streaming data within a unified experience.
To learn more about Informatica’s solutions for streaming data ingestion and real-time decision making, join us for the Meet the Experts webinar, “Ingesting Data to Your Cloud Data Lake or Cloud Data Warehouse,” and read the solution brief.
1,2,3 Gartner, “Adopt Stream Data Integration to Meet Your Real-Time Data Integration and Analytics Requirements,” by Ehtisham Zaidi, Eric Thoo, and W. Roy Schulte, 15 March 2019