Winning in Consumer Packaged Goods with Data Quality
Data Is a High-Value Corporate Asset, and Its Value Is Based on Its Quality
The last two years have seen many industries disrupted, not least companies manufacturing consumer packaged goods (CPG). They have been hit with supply and production challenges, distribution and logistical problems, and shifting consumer buying behaviors. At the same time, CPG companies are investing heavily in digital transformation initiatives to optimize and build resilience into their supply chains, drive product innovation, influence buying behaviors, seize new market opportunities, precisely tailor offerings that fulfill customer needs, and manage risk. All this while maintaining and expanding margins!
While digital transformation initiatives hold much promise, there are significant challenges that can hold you back from achieving your goals. Data proliferation, fragmentation, and decentralization across multiple clouds and hybrid environments is the new normal. According to the World Economic Forum, over 80% of organizations across industries plan to accelerate their digital transformation efforts, yet 70% of digital transformations have failed to achieve their objectives.1
In addition to the wealth of data in the manufacturers’ own systems, they can tap into a vast amount of data from their trading partners that can feed into their decisions on product development, demand planning, procurement, distribution, and sales and marketing. However, all this depends on data; more precisely, high quality data. Let’s look at some examples around product and supplier master data.
The Impact of Poor Product Master Data
Looking at Figure 1, we can see the business impact of poor data quality as it relates to product master data. Each one of these directly correlates with increased costs, lower revenues, time to market, and lower productivity.
Many companies make assumptions about their data and do not ask hard questions about the quality of the data driving their supply chains and the potential impact. One way is to link business policies to potential impact if not achieved, as can be seen in Table 1. The cost of each impact is assessed as part of the business case development, and that assessment also provides a baseline measurement as well as a target for improvement.
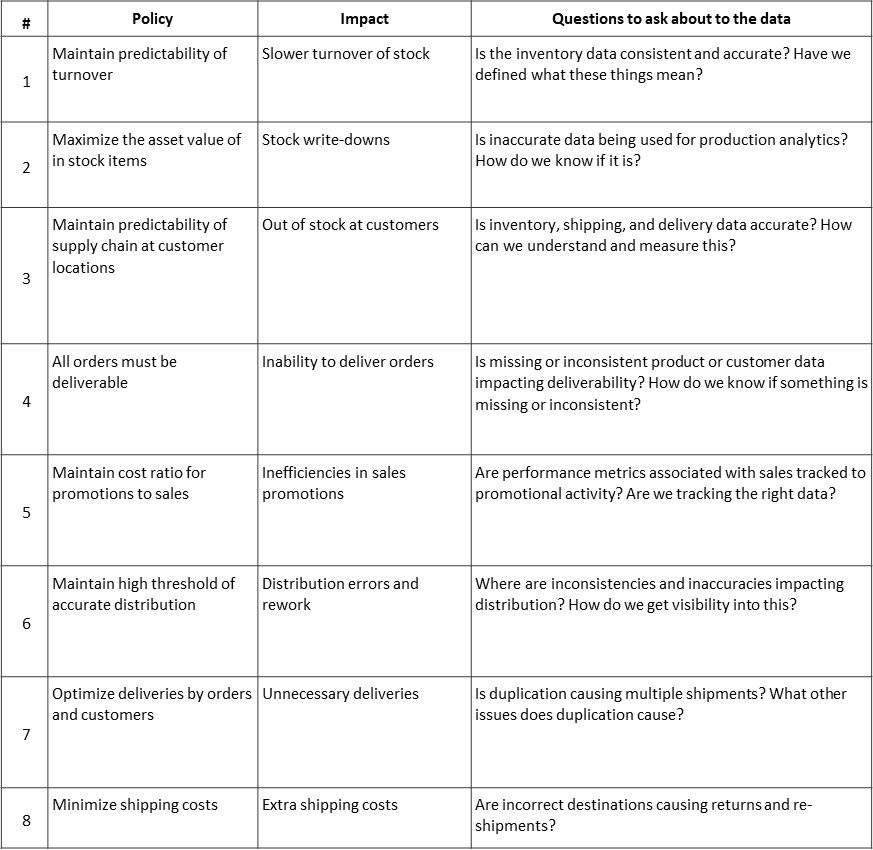
Consider the business policy associated with impact #4: “All orders must be deliverable.” The impact could be incurred because of missing product identifiers, inaccurate product descriptions, and inconsistency across different subsystems, each of which contributes to reducing the deliverability of an order. In turn, assuring that product identifiers are present, product descriptions are accurate, and maintaining data consistency across applications will improve the deliverability of orders.
Gain Critical Insight from Analytics and Reporting
A really big win for data across every enterprise is in analytics: using data-driven insights to better understand your business, your customers, and your processes. With that imperative as a driver, the analytics field has exploded, powered by a new generation of analytics technology.
Many of the benefits in today’s analytics and machine learning come from looking beyond any one data store or departmental silo. It’s about combining many disparate internal and external data sources together to generate insights you couldn’t get from siloed views. It’s about applying machine learning and AI algorithms to recognize behavioral patterns, to identify consumer interests and provide recommendations, and drive innovation. That’s why data quality is so critical to delivering a successful analytics initiative. Bringing together insights across different data sources—from suppliers to retailers to internal systems—requires that the data being combined is authoritative and trusted.
As an overly simple example of the challenges of poor-quality data, Table 2 below depicts examples of typical problems that CPG companies can face.
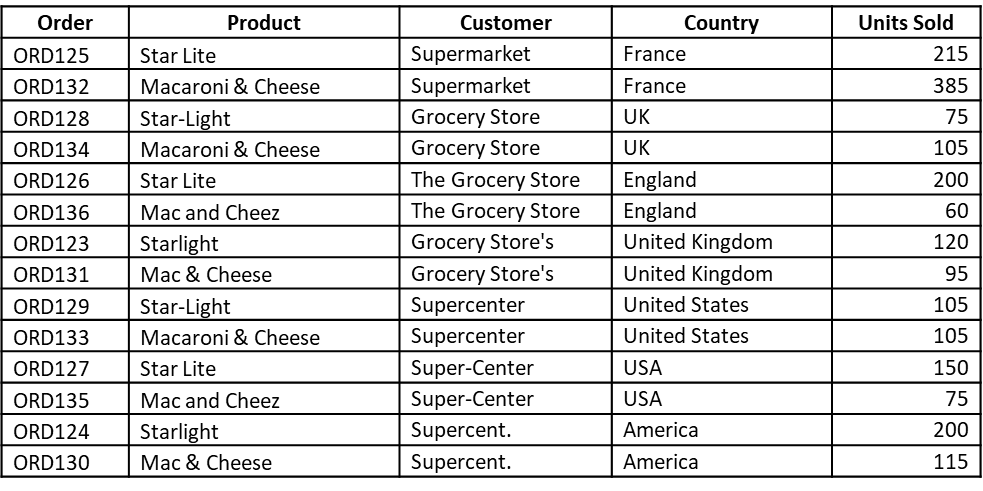
Looking at the table above, how easy is it to identify the top retailer or the top country, or which product sold the most units? Let’s compare how this data might look on a corporate dashboard before and after it is cleansed and standardized.

Now if we add in the multiple variations of a product, such as promotional offers, sizes, and languages, across the huge number of SKUs a typical CPG company manages, the problem becomes exponentially more complex.
Quickly Prepare Data for Enhanced Machine Learning
As CPG companies look to leverage the vast amount of data they have from internal and external sources about their customers, their changing behavior, and a shifting marketplace, they are investing in machine learning and AI.
As the saying goes, “garbage in, garbage out,” which is why data cleansing and standardization are prerequisites for machine learning (ML). Improving the quality of data reduces misleading results and improves model performance. ML relies on high-quality data for quality predictive models.
However, capturing, preparing, and maintaining high quality data for AI and ML is not as easy you think. The data needed to train models comes from many different sources, in multiple formats, and in many cases in huge volumes. And this can throw up a number of data quality issues that impact the AI and ML models. These can be classified using the following data quality dimensions:
- Completeness – What data is missing or unusable?
- Conformity – What data is stored in a non-standard format?
- Consistency – What data gives conflicting information?
- Accuracy – What data is incorrect or out of date?
- Duplication – What data records are duplicated?
- Integrity – What data is missing important relationship linkages?
- Range – What scores, values, and/or calculations are outside of range?
- Data labelling – Is the data labelled with the correct metadata?
This is not an exhaustive list, but these are the most widely used with AI and ML models that I have seen. Other dimensions that customers have used include Exactness, Reliability, Timeliness, Continuity, etc.
Consider this example. A business captures a country detail for use in an ML model. The data it collects produces the following stats:

What does this mean? Either this data did not have proper filters in place up front, or it came from multiple places. The result is inconsistency. And this will impact the results on the ML model.
Data quality is even more important for unsupervised learning, where AI dives into the data to figure things out for itself. (A “black box” approach that makes it very hard to trace data quality issues later.)
Data Is a High-Value Corporate Asset, and Its Value Is Based on Its Quality
Because data is everywhere, data quality is best thought of as a strategic initiative, not just a project team issue or in a silo. Informatica ensures that all key stakeholders in your organization can work together effectively to identify bad data and fix it faster. With the Informatica Intelligent Data Management Cloud, your organization can:
- Proactively cleanse the data for all applications and keep it clean
- Share in the responsibility for data quality and data governance
- Build confidence and trust in enterprise data
Informatica leverages our many years of experience working with customers to identify and resolve their data quality problems. Informatica Cloud Data Quality is part of the Informatica Intelligent Data Management Cloud, so you can quickly identify and resolve data quality issues without any additional IT coding or development. As a result, you can leverage its security, reliability, and backup so you can focus on operational excellence instead of investing in additional infrastructure. Business users can readily specify, validate, and test re-usable data quality rules in a streamlined and collaborative environment.
Start finding and fixing data quality problems today with a free 30-day trial of Cloud Data Quality. To learn more about Informatica data management solutions for CPG organizations, read our white paper, “Capitalize on Emerging Consumer Opportunities in CPG.”