Build a strong data governance foundation to support data-driven decisions with stewardship, data quality, and AI-driven automation.
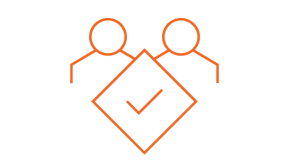
Good decisions require good data
The first step to making sound strategic business decisions is to ensure they are based on reliable, high-quality data. For your employees to trust the data they're using, they also have to trust each other to notice and fix problems with the data before they use it.
A data governance program is designed to do just that.
What is a data governance program?
A data governance program helps organizations evaluate the data they have, where it is located, and how it’s being used so they can improve its quality and reliability. And in today’s high-data-volume world, that also means being able to perform all those tasks at scale. Good governance produces the good data—and the visibility and understanding—you need to make confident decisions.
Better data drives better analytics
A data-driven organization doesn't just give everyone access to data. It makes sure that relevant data is accessible to the appropriate people at the right time. And it makes sure that the data everyone can access can be trusted to deliver meaningful, actionable results. Deciding to democratize data therefore requires the entire organization, from top to bottom and across departments, to commit to protecting data quality. Broad participation in data governance will help teams see it less as rigid control and more as collaboration for enterprise-wide benefits such as:
- More efficient and comprehensive reports that draw on un-siloed data
- Better collaboration between business and IT, thanks to shared responsibility for data quality
- More accurate predictions and planning strategies
- Regular data cleansing and updating
Data stewards play a critical role in taking ownership of data. They determine what data needs to be governed and how, from the highly detailed controls necessary for privacy compliance to more flexible approaches appropriate for exploratory analytics. By augmenting data stewards’ expertise with automated data governance tools that handle common tasks and monitor the health of data over time, organizations can scale the positive effects of optimal data quality. As more users learn they can rely on governed data to drive analytics projects, support for your governance program is likely to grow.
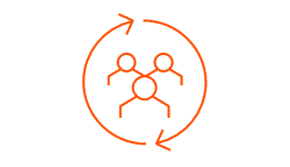
The role of data stewardship
Data stewards are people with specific line-of-business expertise who actively involve themselves in keeping track of where data comes from, how accurate it is, how relevant it is, and how their particular group uses it. Generally, data stewards are people who have a passion for making sure their group's data is of high quality. In a hospital, for example, that may mean an intake nurse from the emergency room, a technician in the radiology department, an administrator in admissions, and a data scientist in IT.
Data governance is essentially an ongoing agreement among these data stewards to share expertise about their own data and collaborate on how best to use it, both within their departments and across functions. They help to curate data and weigh in on how proposed changes will affect their department, providing the human intelligence that enhances analytics to shape better decisions.
Data governance is not a top-down process. It's an ongoing agreement among data stewards to speak up honestly about how changes will affect existing processes across the enterprise. If changing the name of a data field to streamline a key process in one department will break a key process in another department, a data steward must say so and fend off the consequences of what initially seemed like a minor change.
The importance of data quality in decision making
The job of a data governance organization is to prepare data to generate trustworthy results by continually measuring and monitoring it, tracking its quality over time while spotting and correcting errors. This must take place regardless of whether data lives on-premises or in the cloud; data is too important to mission-critical business process for data quality to vary by location.
Data quality becomes increasingly critical as an organization introduces self-service analytics and democratizes access to data. As people are allowed to create their own dashboards and use data for their own analyses, the data governance program must ensure that the data is reliable. One unsuccessful decision based on flawed data can destroy trust in the entire decision-making process for months or even years.
The data governance team protects data quality by:
- Agreeing on common policies and procedures
- Creating shared definitions and terminologies
- Ensuring that everyone has access to the same, consistently high-quality data
Without consensus and consistency around these three factors, data quality and consistency will lag, and end users will arrive at different conclusions, make different decisions, and try to steer the company in different directions, with potentially disastrous results.
How AI and machine learning help data governance scale across the enterprise
The volume, variety, and velocity of data flooding into organizations every second is too much for human data stewardship to handle by itself. Data governance tools that incorporate artificial intelligence (AI) and machine learning make it possible to automate common and repetitive tasks for more accurate governance in less time—and with less chance of errors.
The power of AI/ML helps automate the process of finding data, measuring its quality, and locating the right people to help govern it. It can generate, measure, and monitor data quality based on business definitions, allowing data stewards to visualize data quality and find the best data sets for a specific project. It can detect similar data across thousands of records to complete tasks in days that once took months, such as recognizing and cleansing address information regardless of data source, then enriching that data with additional attributes. It can derive structure from messy device and log files, making them easier to understand and work with. AI and machine learning are also invaluable for detecting outliers and anomalies that might indicate human error, deliberate data contamination, or other risks to data quality and regulatory compliance efforts.
Governance and the data-centric business
Today's organizations don't simply use data: they can't function without it. Traditional approaches to data governance don’t have the speed or scale necessary to provide the consistently clean, trustworthy information that today’s systems and business leaders demand. Companies need a cross-functional data governance team, supported by a data management platform that uses data, metadata, and AI and machine learning to support next-generation analytics and facilitate confident decision making across the entire organization.
Learn more about data governance and business benefits in our Reimagine Data Governance webinar series: