Learn why big data privacy is a critical component of any data management initiative, and how to develop modern big data privacy and protection strategies.
What is big data privacy?
Big data privacy involves properly managing big data to minimize risk and protect sensitive data. Because big data comprises large and complex data sets, many traditional privacy processes cannot handle the scale and velocity required. To safeguard big data and ensure it can be used for analytics, you need to create a framework for privacy protection that can handle the volume, velocity, variety, and value of big data as it is moved between environments, processed, analyzed, and shared.
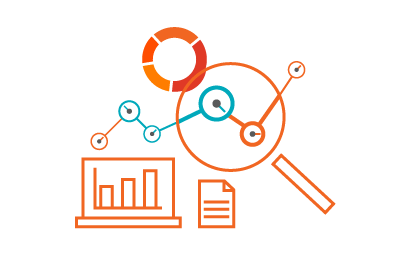
Big data includes big privacy concerns
In an era of multi-cloud computing, data owners must keep up with both the pace of data growth and the proliferation of regulations that govern it—especially regulations protecting the privacy of sensitive data and personally identifiable information (PII). With more data spread across more locations, the business risk of a privacy breach has never been higher, and with it, consequences ranging from high fines to loss of market share.
Big data privacy is also a matter of customer trust. The more data you collect about users, the easier it gets to "connect the dots:" to understand their current behavior, draw inferences about their future behavior, and eventually develop deep and detailed profiles of their lives and preferences. The more data you collect, the more important it is to be transparent with your customers about what you're doing with their data, how you're storing it, and what steps you're taking to comply with regulations that govern privacy and data protection.
The volume and velocity of data from existing sources, such as legacy applications and e-commerce, is expanding fast. You also have new (and growing) varieties of data types and sources, such as social networks and IoT device streams. To keep pace, your big data privacy strategy needs to expand, too. That requires you to consider all of these issues:
What do you intend to do with customer and user data?
How accurate is the data, and what are the potential consequences of inaccuracies?
How will your data security scale to keep up with threats of data breaches and insider threats as they become more common?
Where is your balancing point between the need to keep data locked down in-place and the need to expose it safely so you can extract value from it?
How do you maintain compliance with data privacy regulations that vary across the countries and regions where you do business, and how does that change based on the type or origin of the data?
How do you maintain transparency about what you do with the big data you collect without giving away the "secret sauce" of the analytics that drive your competitive advantage?
Predictions for big data privacy: What to expect
Prediction 1: Data privacy mandates will become more common.
As organizations store more types of sensitive data in larger amounts over longer periods of time, they will be under increasing pressure to be transparent about what data they collect, how they analyze and use it, and why they need to retain it. The European Union's General Data Privacy Regulation (GDPR) is a high-profile example. More government agencies and regulatory organizations are following suit. To respond to these growing demands, companies need reliable, scalable big data privacy tools that encourage and help people to access, review, correct, anonymize, and even purge some or all of their personal and sensitive information.
Prediction 2: New big data analytic tools will enable organizations to perform deeper analysis of legacy data, discover uses for which the data wasn't originally intended, and combine it with new data sources.
Big data analytics tools and solutions can now dig into data sources that were previously unavailable, and identify new relationships hidden in legacy data. That’s a great advantage when it comes to getting a complete view of your enterprise data—especially for customer 360 and analytics initiatives. But it also raises questions about the accuracy of aging data and the ability to track down entities for consent to use their information in new ways.
The key to protecting the privacy of your big data while still optimizing its value is ongoing review of four critical data management activities:
Data collection
Retention and archiving
Data use, including use in testing, DevOps, and other data masking scenarios
Creating and updating disclosure policies and practices
Companies with a strong, scalable data governance program will have an advantage when assessing these tasks—they will be able to accurately assess data-related risks and benefits in less time and quickly take more decisive action based on trusted data.
Developing big data privacy and protection strategies
Traditional data security is network- and system-centric, but today's multi-cloud architectures spread data across more platform-agnostic locations and incorporate more data types than ever before. Big data privacy can't be an afterthought. It must be an integral part of your cloud integration and data management strategy:
You must define and manage data governance policies to clarify what data is critical and why, who owns the critical data, and how it can be used responsibly.
You must discover, classify, and understand a wide range of sensitive data across all big data platforms at massive scale by leveraging artificial intelligence and machine learning tools to automate controls; then, you can use that information to develop and implement intelligent big data management policies.
You must index, inventory, and link data subjects and identities to support data access rights and notifications.
You must be able to perform continuous risk analysis for sensitive data to understand your risk exposure, prioritize available data protection resources and investments, and develop protection and remediation plans as your big data grows.
You need automated, centralized big data privacy tools that integrate with native big data tools like Cloudera Sentry, Amazon Macie, and Hortonworks Ranger to streamline and facilitate the process of managing data access, such as viewing, changing, and adding access policies.
You need fast and efficient data protection capabilities at scale, including dynamic masking for big data as it's put into use in production and data lakes, encryption for big data at rest in data lakes and data warehouses, and persistent masking for big data used in non-production environments like development and analytics.
You must measure and communicate the status of big data privacy risk indicators as a critical part of tracking success in protecting sensitive information while supporting audit readiness.
Learn more about how Informatica makes big data privacy an integral part of data governance and compliance.
Big data privacy resources
What is Big Data Privacy: A summary overview of privacy for big data
Practical approaches to big data privacy over time: A study of best practices for protecting data from long-term privacy risks
Big Data Governance: 4 steps to scaling an enterprise data governance program
Informatica Big Data Security: Discover Informatica's approach to big data privacy challenges
“Unleash the Full Power of Data: Accelerating Self-Service, High-Value Data for Deeper Insights”: Read the ebook and discover five critical steps to create a cloud-based data lake