What Is Data Preparation?
Data preparation is the process of cleaning, standardizing and enriching raw data to make it ready for use in analytics and data science. Data analysts struggle to get relevant data in place before they start analysis. In fact, data scientists spend more than 80% of their time preparing the data before using it in machine learning (ML) models. This is the 80/20 rule: Data analysts and data scientists spend only 20% of their time on actual business analysis. The rest is spent on finding, cleansing and organizing data.
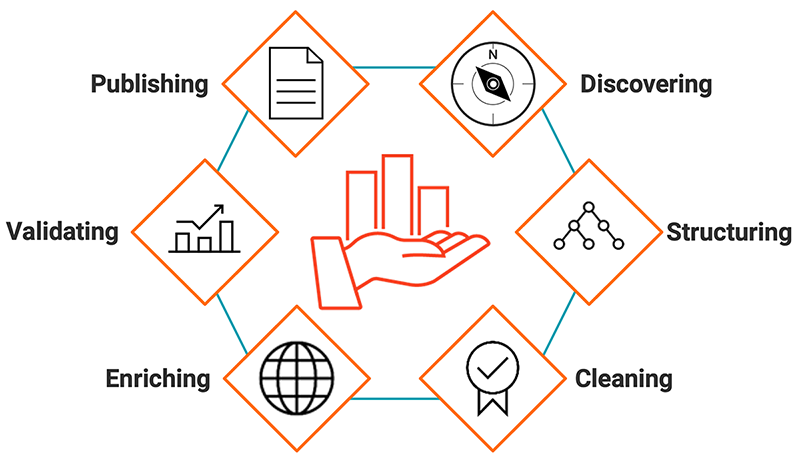
Data preparation takes place over six steps from data discovery through publishing.
Data preparation solves this through an agile, collaborative and self-service approach. It flips the 80/20 rule to a company's advantage. It enables IT departments to offer self-service capabilities on their data assets. And it empowers data analysts to:
- Discover the right data asset
- Prepare the data
- Apply data quality rules
- Collaborate with others
- Deliver business value in less time
Why Is Data Preparation Important?
Today's enterprises generate data daily through customer interactions, business processes and transactional activities. Based on these trends, we see that almost everyone can get access to the same computing power. Most organizations have access to the same set of ML algorithms, too. The only difference is the data and how enterprises use it to make business decisions. The data that enterprises store in different places, be it on-premises or in the cloud, is messy and incomplete. Because of this, employees cannot use this data to run their analytics and data science projects. This is where data preparation becomes critical.
Data Preparation for Analytics
Data preparation is a challenge as organizations embark on data and analytics initiatives. In a typical data infrastructure, data is distributed across data lakes, data warehouses, databases, flat files, XML files, CSV files, audio files, etc. This makes it tough to find, clean, transform and share data for driving advanced analytics use cases.
An AI-powered data preparation tool with an easy-to-use interface can help make data analysts more productive by making it easier to quickly access the data they need. This saves time and money.
Data Preparation for Machine Learning
Using hand coding and manual approaches like Excel spreadsheets for data preparation is time-consuming and redundant. Organizations have realized that ML and AI are critical for generating business value and making data-informed decisions.
ML techniques are used across every industry. They are used for a variety of solutions involving virtually all data types – structured, semi-structured and unstructured data.
High-quality, trusted and governed data is key to the success of any ML initiative. Many ML projects fail due to the use of untrusted data in training datasets. This makes it a “garbage in and garbage out” scenario. Due to these reasons, data preparation is essential for ML projects.
But the manual data preparation process can become tedious due to the high volume and variety of data. Data scientists need high-quality training data to train the ML models. You need to infuse intelligence and automation into the data preparation process. You also need to provide the correct dataset recommendations and clean and transform the data for ML consumption. Data analysts and data scientists can improve their efficiency by focusing on building models rather than investing time and effort in preparing data to train the model.
What Is Self-service Data Preparation?
Historically, data preparation was tough for analysts to do themselves. So, it was often limited to IT through a complex hand-coding process. Plus, IT doesn't have the necessary business context of the data to prepare the data efficiently.
A self-service data preparation tool allows data consumers to search for relevant datasets and make them ready for use. Data consumers will now be empowered citizen integrators. An intuitive, self-service environment from data profiling to publishing the datasets will save time for everyone.
Informatica Data Prep Capabilities
Informatica’s cloud data preparation capabilities allow data scientists, data analysts and citizen data integrators to conduct low-code/no-code, agile data preparation on cloud data warehouses and cloud data lakes to drive self-service analytics and AI/ML use cases. It is a service of the Informatica Intelligent Data Management Cloud™ (IDMC), which is designed for hybrid and multi-cloud environments. Here are six ways Informatica cloud data preparation capabilities help meet data needs:
- Data catalog:
An embedded data catalog helps data scientists and data analysts discover the data they need. It also helps them better understand the data by looking at the metadata. The data lineage capability can help show how any particular dataset is related to other data.
- Data profiling:
Cloud data preparation helps automate end-to-end data profiling to identify data anomalies, outliers and frequency distribution. It also helps you better understand the shape and size of the data, which helps you determine what to do with it.
- Data transformation:
A low-code/no-code user interface helps data analysts prepare data for analytics or data science consumption with no hand coding.
- Data governance:
Cloud data preparation uses embedded governance capabilities in IDMC. This enables data engineers and data analysts to enforce governance and compliance policies on the prepared data models.
- Data enrichment:
Cloud data preparation formalizes third-party data such as customer geographical data, sensor data and customer segment data within the data model. This drives customer 360 use cases.
- Operationalization of data and AI models:
Cloud data preparation enables DataOps teams to operate in an agile and iterative manner. It also provides the ability to operationalize the models.
Industry Use Cases for Data Prep
- Healthcare:
Data preparation can enable healthcare and pharma companies to speed research and development, accelerate drug discovery and deliver breakthrough therapies faster.
- Insurance:
Data preparation supports use cases in the insurance sector like customer 360, risk management and underwriting.
- Manufacturing:
Data preparation helps drive use cases in the manufacturing industry such as supply chain optimization, asset management and operational intelligence.
- Public sector:
Data preparation also supports use cases like cybersecurity, improving citizen experience and case management.
Next Steps
Interested in learning more? Get early access to this new IDMC capability. To participate in our data prep preview program, email dataprep.preview@informatica.com.