CI/CD Pipeline: A Continuous Integration Approach to Data Pipelines
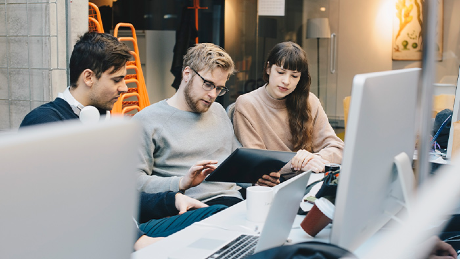
When it comes to modern software development, it’s not surprising that companies have a need for speed. On any given day, there’s so much software in play and so little time to manage it. Organizations strive to release the best software versions as fast as possible. This is all part of the modern software delivery process. That’s why, in the world of big data, agile software development is the new normal. But what happens when you develop software too quickly? It can mean sacrificing quality, security and compliance. DevOps and continuous integration & continuous delivery (CI/CD) are proven capabilities that help companies maintain a healthy balance between agility and quality. DevOps is synonymous with “continuous.” It’s all about continuous integration, continuous delivery and continuous deployment. With DevOps, automated releases go out many times a day. If you’ve implemented DevOps for software development, you have likely experienced the cultural changes that DevOps brings to an organization. And you may be one step ahead when it comes to bringing DevOps to your data pipeline. Here are ten benefits for taking a DevOps and continuous integration approach to your data pipeline:
1. Reduce challenges with data integration
Continuous software delivery requires an intelligent approach to data integration and data management. The process starts with unit testing. Then it extends to QA, performance, acceptance testing and other stages. You can automate your team’s testing at each level of data pipeline development with CI/CD.
2. Deliver value faster and at scale
Data processing can benefit from AI-powered automation. Integrated CI tools help you automate your data pipeline for continuous deployment. CI/CD tools help ensure better data quality and faster release cycles. Your team will be more productive thanks to the ability to do things like store reusable components. CI/CD helps ensure better data quality for enhanced business value.
3. Meet enterprise-level SLAs
CI/CD tools help you deliver on your service level agreements (SLAs). Apply automated data testing at every step, reduce error rates and see fewer bugs escape to production. DevOps methods allow any data engineer to modify the data pipeline. It also ensures that only quality jobs pass through the drill.
4. Quality and reusability with data artifact lifecycle management
It’s powerful when you can treat data pipelines as product. Software development teams can leverage this capability at all stages including:
- Requirements analysis
- Design
- Development
- Automated building
- Build test
- Implementation
- Documentation
- Evaluation
With data lifecycle management, DevOps methods serve as a guardrail that makes it easier to test, release and reuse data all the way to the production environment. A continuous feedback mechanism helps data engineers and the DevOps team to:
- Optimize data delivery pipelines
- Improve code quality
- Enhance performance and speed
- Create and update data objects like mappings, task flows and other artifacts
- Reuse objects later for no-code/no-build data integration activities
5. Seamless collaboration
One way DevOps methods and CI/CD pipelines speed up releases is by enabling teams to work in parallel. With check-in and check-out options for code, multiple team members can work independently on the same objects, with fewer conflicts. An automated feedback mechanism eliminates friction between different personas like data engineers, integrators and operators. With real-time feedback, data engineers can iterate faster. They can leverage automation to more easily optimize operational overhead. This makes the CI/CD pipeline a win-win for all!
6. Version control and user independence
Tracking software versions encourages transparency and ownership. This reduces avoidable concerns about who else is working on specific versions, and other dependencies. Role-based privileges and permissions ensure data pipeline reliability and security.
7. Standardization
DevOps drives standardization in terms of process, toolchain and the framework in general. Loosely coupled modular systems or applications can be used as building blocks. This makes it easier to adapt to future technology or process change. At any time, you can add another layer of testing to optimize your data pipeline. With DevOps methods, you can provision those changes — something you can’t do with hand-coding. A standard process helps your team track and adapt to a changing business landscape.
8. Enable experimentation
DevOps encourages agile experimentation. It lets you roll back to the previous data management version any time. This is critical when the new version is not working out. Developers can also try out new technologies and tasks. Gatekeepers can maintain quality at every step along the way.
9. Monitoring
With an automated alert and response system, it’s easier to troubleshoot and monitor CI/CD pipelines. If there’s a break or issue in the workflow, developers can quickly rectify the situation without bothering the operations team.
10. Getting ready for DataOps, MLOps and AIOps
Depending on your organization’s data maturity level, you can apply the knowledge of DevOps to customize data products and operationalize machine learning (ML) models and artificial intelligence (AI) projects in the future.
A Real-World Example of a Continuous Integration Approach to Data Pipelines
Let’s take the example of our customer, Guy Carpenter, a leading global risk and reinsurance specialist. This use case focuses on their DevOps approach in a hybrid cloud landscape. While moving through multiple release stages, the company is able to streamline and automate their data processes. In the development stage, they write a task and conduct unit testing. Once committed, the code moves into the system integration testing environment. Next it passes through the QA testing phase. After that, it goes to pre-production performance or user acceptance tests. And finally, the software moves into production. Thanks to automation, the whole process takes care of itself. This brings agility, productivity and efficiency to enhance business results. This is true no matter where you are in your pipeline building, testing and deploying process.
To learn more, watch this video, “How Guy Carpenter brought CICD automation to their iPaaS using Azure DevOps.”
How Informatica Can Help
Informatica cloud-native data integration solutions provide out-of-the box CI/CD capabilities. They enable you to break down silos across development, operations and security to deliver a consistent experience across the development lifecycle.
Watch this video to learn more about CI/CD pipelines with zero-code and zero-build data integration. You can also reach out to us anytime with questions or to explore next steps.