Big Data in Healthcare: Driving Digital Transformation
I spend much of my time meeting with healthcare executives and discussing how they can transform their organizations using data. Perhaps unsurprisingly, these discussions often start with a focus on the discrete data captured in electronic health records or the codified content of claims and other transaction applications for payers.
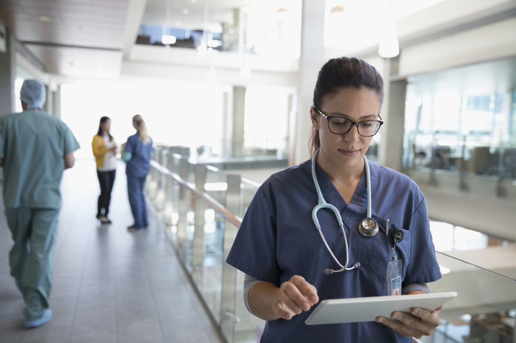
However, focusing on just the discrete data available from healthcare transaction applications ignores the huge potential of information that is readily available, yet under-utilized: big data. And I’ll suggest that it is the insights in healthcare big data that will drive enormous transformations in efficiency, quality of care, and patient/provider engagement.
What is big data in healthcare?
Healthcare big data is the immense volume and variety of health data generated by health-related systems and devices, including but not limited to:
- Electronic health records, medical imaging, patient portals, and virtual assistants
- Genomic sequencing, pharmaceutical research, and treatment development and testing
- Medical device and biometric data, diagnostic equipment, wearable devices (like activity trackers and Apple Watches), smartphone apps, and equipment tracking sensors
- Payer records, public records, risk assessments, service reviews, government agencies, and search records
The rise of the Internet of Things (IoT), digital and cloud technologies, and tech-savvy patients are all contributing to massive data growth in the healthcare industry—a 36% compound annual growth rate between 2018-2025, according to the IDC Data Age 2025 report.
Big data examples in the healthcare industry
Here are some of the greatest opportunities for big data in healthcare.
Mining nuance and insight from text notes in electronic health records
Electronic health records (EHRs) capture the clinical notes from a patient’s physicians, nurses, technicians, and other care providers. These notes are a treasure trove of unstructured digital information that would be highly valuable to mine using natural language processing (NLP) and other techniques. They provide far richer nuance and context about a patient’s medical history, diagnoses, treatment plans, test results, and other details than codes and other reference data—so ubiquitous across healthcare—ever could. HealthITAnalytics published a nice article highlighting some examples of NLP applied to text notes: Massachusetts General Hospital used NLP to reliably derive social determinants of health information, and Beacon Health Options is using machine learning and NLP to identify patients at risk of “falling through gaps in the healthcare system.”
Using artificial intelligence and machine learning on medical images.
The healthcare industry moved to digital imaging decades ago, and for years the focus was simply on managing the huge volume of storage required for the images and building the network capacity to ship the large image files around the network. This evolved into using digital image processing techniques to construct 3D models of the imaged structures which was a huge advance. But one of the hottest areas with the exciting potential to impact efficiency and quality of care is in applying artificial intelligence (AI), machine learning (ML), and big data processing power to medical imaging.
According to an article published in Nature Reviews Cancer and available in the National Center for Biotechnology Information (NCBI) resource center, there is much more imaging data available than there are available and trained readers to evaluate it. In addition, “the decline in imaging reimbursements has forced healthcare providers to compensate by increasing productivity. These factors have contributed to a dramatic increase in radiologists’ workloads. Studies report that, in some cases, an average radiologist must interpret one image every 3–4 seconds in an 8-hour workday to meet workload demands. As radiology involves visual perception as well as decision making under uncertainty, errors are inevitable—especially under such constrained conditions.”
By integrating AI/ML into the imaging workflow, you can add intelligent automation capabilities to pre-screen images and flag potential issues for trained radiologists—helping to increase efficiency and reduce both errors and need for manual input.
Analysis of genomic and proteomic population data sets.
The volume of genomic and proteomic data being generated—and the potentially life-changing discoveries that could be gained from that data—is astounding. We can now sequence an individual’s complete genome in hours rather than years, and for less than $1,000.
Looking at genomic and proteomic data for just a single individual creates a huge data set. When you consider this data across an entire population, the big data opportunity couldn’t be clearer: this data is gargantuan in volume, and individual variations across populations is intriguing. I won’t pretend to understand all of the potential applications of this enormous and complex big data, but even to a casual bystander the analytic opportunities are life-changing. We routinely see new drugs and therapies that include genetic testing as a component to assess potential efficacy, as well as the identification of genetic patterns to predict cancer and other conditions with sufficient accuracy to be clinically useful.
One example of the power of genomics data is illustrated by the 100,000 Genomes Project. The project was established to sequence 100,000 genomes from around 85,000 National Health Service (NHS) patients affected by a rare disease or cancer. It completed its participant recruitment in 2018 and noted its 100,000th sequence in December 2018. According to the organization, it has uncovered actionable findings “in 1 in 4/1 in 5 rare disease patients, and around 50% of the cancer cases contain the potential for a therapy or clinical trial.”
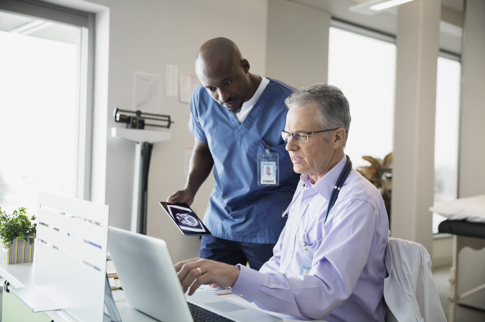
Leverage insights from streaming data and devices
EKGs, intravenous pumps, respirators, and myriad other medical and health monitoring devices—as well as wearable personal devices like Apple Watches—generate huge volumes of data. In a clinical setting, this data is traditionally used for real-time monitoring but then discarded as being too voluminous and perceived of no/low value. Consumer device data is frequently captured but in proprietary silos that are not part of the larger healthcare data ecosystem.
But capturing and analyzing this streaming data can provide a wealth of information for patients and providers. Healthcare organizations can use it to detect patterns that indicate subsequent bad events, such as sepsis in the ICU. Predictive analytics can detect warning signs that enable providers to intervene early enough to head off poor outcomes—saving lives as well as reducing liability and costs of patient care. The Mayo Clinic’s submission for approval to the FDA of a “bloodless blood test” uses AI against device-collected electrocardiogram (ECG) data to predict the potassium level in blood, instead of an invasive medical test (for example, a venipuncture blood draw). This idea of replacing an invasive test—such as a blood draw that is expensive, requires special skills and facilities, and requires the patient to be physically present—with a readily available, non-invasive, essentially free and portable measure from a smart watch, is an example of a radically more connected healthcare future enabled by the IoT, big data, and AI.
Challenges of implementing big data solutions in the healthcare industry: What’s taking so long?
Healthcare has long recognized that the time required to take innovations from basic research to clinical practice (“bench to bedside”) is exceedingly long. While explainable and perhaps justifiable in the past because of the relative scarcity of facilities able to do the basic research and invention of new knowledge leading to clinical trials, I believe big data opens an entirely new avenue of innovation in healthcare.
With big data and the widespread availability of affordable processing power and storage, a far greater number of organizations and knowledgeable individuals have the opportunity to look for novel healthcare insights. This does not mean we should not proceed with caution, but we cannot ignore the fact that potential avenues of innovation have expanded by orders of magnitude with the widespread availability of big data in healthcare.
Equally exciting is the potential for some atypical actors who have phenomenal big data skills (think Amazon, Google, Apple, or Microsoft) to change the healthcare landscape by partnering with expert healthcare organizations to derive compelling, actionable insights from healthcare big data.
Now is the time for healthcare organizations to embrace digital transformation and the potential of big data. The opportunity is too great to ignore and the potential to fundamentally change lives for the better is simply too compelling.
Learn about our healthcare analytics solutions and see how Informatica can help you take advantage of big data, AI, and other data integration and data management technologies to transform your organization.