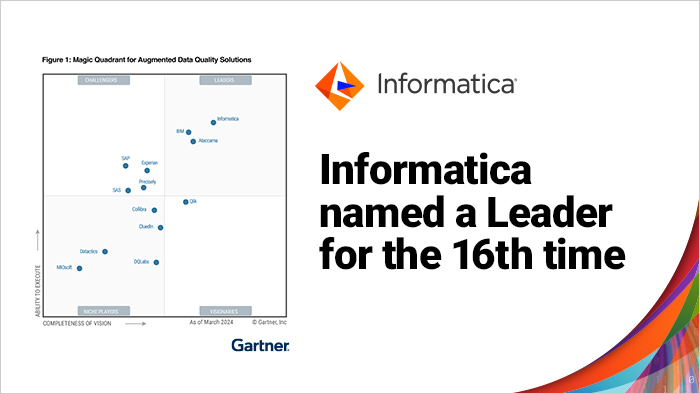
Data Governance & Data Privacy
Mar 11, 2024
Data Governance & Data Privacy
Mar 11, 2024
Recommended for you
Trending Now
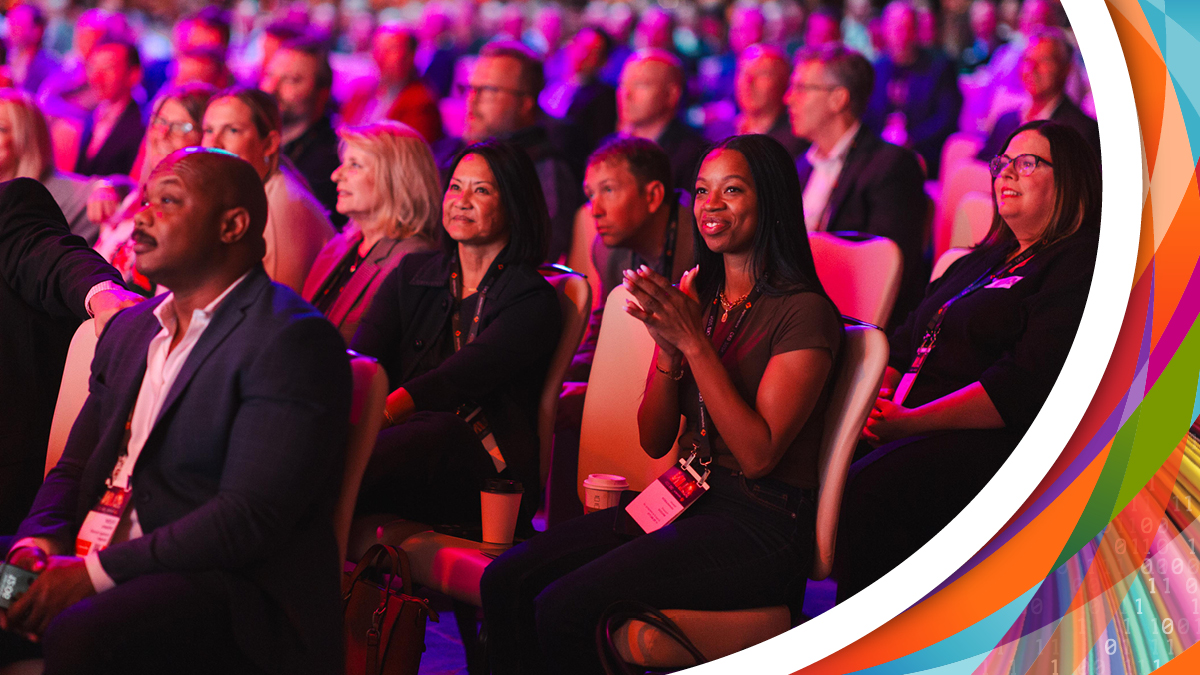
Life@INFA
Mar 08, 2024
Life@INFA
Mar 08, 2024
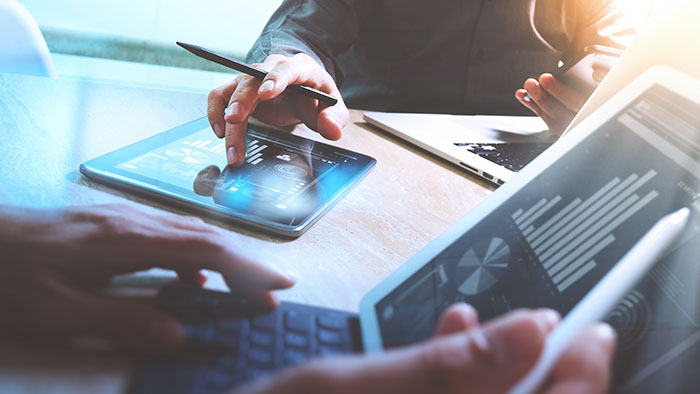
Cloud Data Management
Feb 23, 2024
Cloud Data Management
Feb 23, 2024
Data Governance & Data Privacy
Mar 11, 2024
Data Governance & Data Privacy
Mar 11, 2024
Life@INFA
Mar 08, 2024
Life@INFA
Mar 08, 2024
Cloud Data Management
Feb 23, 2024
Cloud Data Management
Feb 23, 2024