5 Keys to Operationalizing Data Analytics in the Cloud
Enterprises across the globe are analyzing large datasets to uncover patterns and valuable insights, which in turn inform their decisions. These business insights and decisions result in several benefits for enterprises such as: lower costs, improved operational efficiency, new revenue streams, fraud detection, increased customer engagement and satisfaction, competitive advantage, and more.
Analytics at Cloud Scale
Traditional data analytics that helped organizations drive business intelligence and reporting were slower, less efficient, and expensive to maintain. Now with big data analytics, your data users—such as data scientists, and data analysts—can tap into structured, semi-structured, and unstructured data at petabyte-scale and at various speeds (batch and real time). Data to be analyzed is typically hosted in a cloud data lake such as Amazon Web Services (AWS) S3, Microsoft Azure Data Lake Store (ADLS), Databricks Delta Lake, etc. and often pushed downstream into cloud data warehouses, AI/ML workbenches, and/or analytics/visualization tools.
To capitalize on cloud analytics across your enterprise, you need to take a systematic approach to analytics. That is, you need to operationalize analytics.
What Is Analytics Operationalization?
Analytics operationalization is the process of bringing the right data, at the right time, for the right users —all in a repeatable and collaborative fashion, where the data can be trusted for business insights and actions.
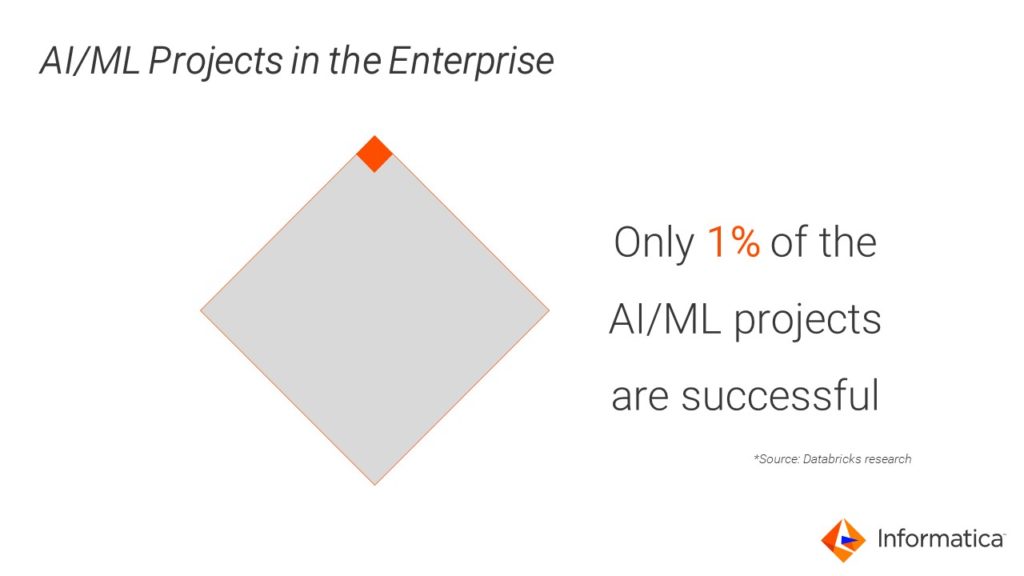
Why Operationalize Analytics?
Analytics need to be operationalized for several reasons. Currently, analytics:
- Take too long to build and realize benefits. Most analytics projects take 6 to 12 months to go to production and gain enterprise-wide adoption.
- Lack collaboration among data engineers for analytics data pipeline building and sharing. There’s also a lack of collaboration between business and IT, that is, data analysts, data scientists, and data engineers.
- Never go into production. According to research, 60% of the analytical projects developed by organizations never are operationalized, or put into use.
- Not in scaled production. Many analytics projects are never deployed at large scale. McKinsey research indicates only 8% of companies successfully scale their analytics.
- Lack trust and governance. Without proper data quality or data protection, you can’t reliably use such insights or inform actions.
- Are rarely successful. In the case of AI/ML and predictive analytics projects, Databricks’ research suggests only 1% are successful
- Aren’t complete. Many analytics projects don’t handle a variety of data, so they are incomplete. Without streaming data processing, analytics projects can result in decisions made without complete data, and consequently, they don’t produce the desired results.
As a result, enterprises across industries and geographies are falling short of converting insights into action.
5 Keys to Operationalizing Analytics at Cloud Scale
People and process are two of the three key pillars of analytics operationalization. Technology, specifically data management, is the third key pillar to succeeding with analytics at cloud scale, on time, and enterprise wide.
Here are five keys to making sure you’re able to operationalize analytics:
- Get the Right Data. Before you embark on an analytics or AI project it’s important to find and access all the data sources you need and ingest the necessary data into your cloud data lakes. Informatica Enterprise Data Catalog (EDC) and Cloud Mass Ingestion (CMI) offer a simple 5-step wizard to accomplish this.
- Accelerate Development. You can speed up the development of your analytics data pipeline with the help of a zero-code, easy-to-use visual interface to rapidly build data transformation, real-time data processing, and data wrangling functions. Informatica products that help here are Informatica Data Engineering Integration (DEI), Data Engineering Streaming (DES), and Enterprise Data Preparation (EDP).
- Ensure Collaboration and Automation. Enhance your data users’ productivity with analytics data pipeline recommendations, cross data pipeline categorization, streaming data lineage, and dataset and recipe recommendations. Informatica DEI, DES, and EDP are all powered by the metadata-driven artificial intelligence in the Informatica CLAIRE™ engine.
- Promote Data Governance. Deploy prebuilt data quality rules so you can easily handle the scale of big data to improve quality across the enterprise. Use relevant, accurate, clean, and valid data to operationalize your machine learning models. Informatica Data Engineering Quality (DEQ) and Data Engineering Masking (DEM) help ensure trusted, high-quality data for your analytics projects.
- Follow Deployment Best Practices. Follow the CI/CD process to deploy big data analytics pipelines, and further it with scaled production. Informatica DEI and EDP products are essential here. And Informatica Operational Insights is key to monitoring big data resources (watch the demo video to see why).
Learn more:
Join us at these upcoming events to see how Informatica can help operationalize your analytics at cloud scale:
- Join the Data for AI and Analytics Virtual Summit in North America or EMEA
- Join the Meet the Experts webinar: What's New in Data Engineering Integration