How AI and Its Generative Capabilities Are Reshaping Data Management
Hollywood has been fascinated with AI narratives for over four decades. From H.A.L in 2001: A Space Odyssey to the operating system in HER and J.A.R.V.I.S. in Ironman, these movies often make us wonder about the potential impact of systems like J.A.R.V.I.S. on humans and the ways they could improve our quality of life.
While we may not have AI systems governing cities just yet, the strides made in AI have been impressive. AI and data management go hand in hand, and businesses that embrace AI-enabled data management can automate numerous tasks within the data pipeline, from ingestion to governance. The user experience for data management solutions has significantly improved, thanks to the reduction in manual labor required to build data pipelines, maintain data quality and enforce data governance. These enhancements are made possible by incorporating AI/ML capabilities. In this article, we will explore some areas where AI is making a substantial impact on data management.
Self-Integrating Systems
According to a recent report, data professionals spend a large portion (38%) of their time on data preparation and cleaning rather than more advanced tasks such as selecting, training and deploying models. It also highlights that 63% of commercial respondents are concerned about the shortage of data professionals1.
Manually building and debugging data pipelines is a highly time-consuming process. It entails setting up source and destination points, establishing connections between systems and adhering to schema requirements before initiating the data flow. However, business requirements may change, leading to further complications. In addition, data pipelines may need to be taken down for upgrades or to correct data source errors, which can result in repetitive work for data engineers.
AI for the Win
AI can alleviate much of the trouble associated with managing data pipelines, such as:
- Infusing intelligence into data integration and streamlining all data management tasks.
- Automating the generation of crucial data pipelines.
- Handling unstructured, semi-structured and hierarchical data sources with data management solutions powered by AI.
- Automatically implementing masking, cleaning and standardization rules.
- Supporting the joining, union, normalization and denormalization of data sources. This results in an autonomous data integration experience.
A self-integrating system understands what integration method is suitable for the type of pipeline and workload. As illustrated in Figure 1, CLAIRE, Informatica’s AI-powered engine for data management, navigates workload, cost of execution and sources involved. It is able to target and decide on a mechanism like ELT or source transformation or a real-time streaming load.
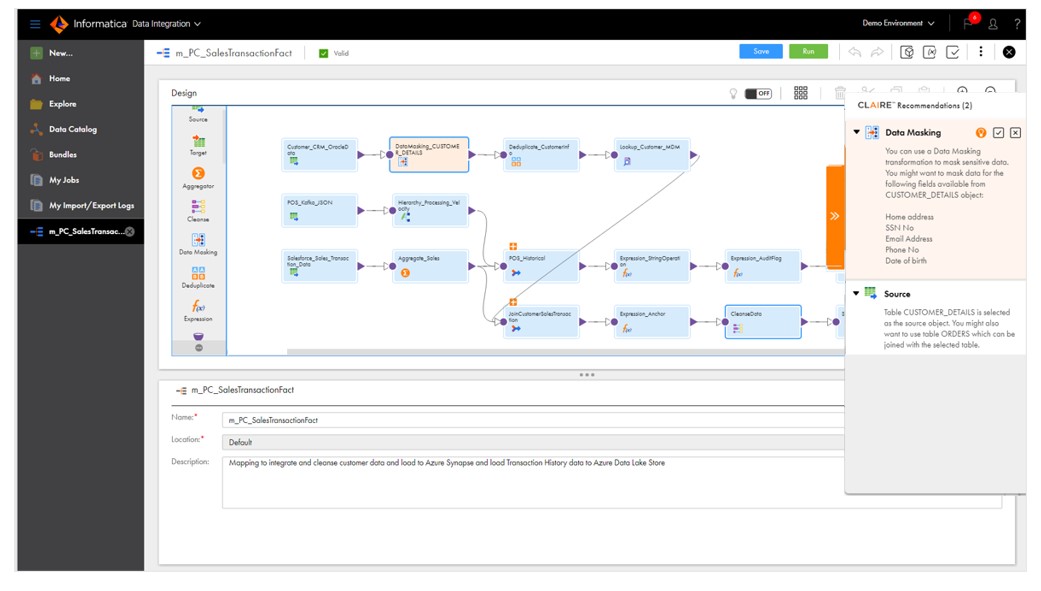
Data Governance: Automatically Assessing Data Quality
Data governance is a critical component of maintaining data quality throughout a system. The data governance office must ensure that the data is complete, accurate, consistent and valid to support business operations. As data governance implementations grow, assessing quality for a growing number of systems and fields becomes increasingly time-consuming. However, with the assistance of the Informatica Cloud Data Governance and Catalog and Informatica Cloud Data Quality, your CLAIRE AI copilot can automate the application of data quality measurements across the enterprise, saving thousands of hours of work. Automated data quality measurements are the way forward to ensure that your data is trustworthy and good enough to support your business operations.
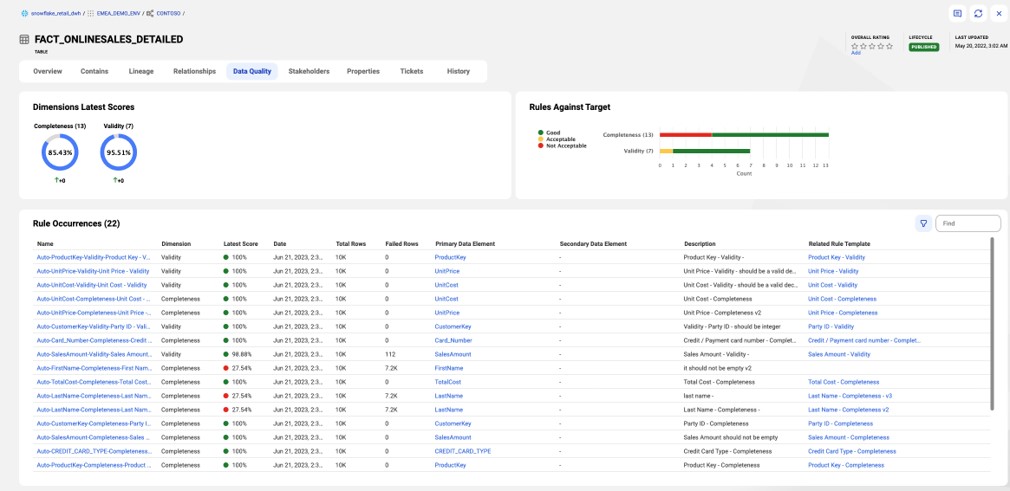
CLAIRE combines knowledge of critical business elements, portable and executable data quality rules and metadata details from physical data assets to generate data quality rule execution jobs in Cloud Data Quality (depicted in Figure 2). It saves thousands of manual labor hours by linking the results to the correct dashboards and creating aggregated views for consumption by the governance office. CLAIRE also helps automatically assess new physical assets for quality and discovers new domains using named entity extraction or a classifier in data quality rules.
ML/NLP-Assisted Data Quality Rule Creation
Data quality is a crucial aspect of any data governance program, especially in larger implementations with numerous data quality rules. To help data stewards identify the correct rules to use, CLAIRE, your AI copilot, can assist in identifying rules and generating any missing ones. If you're a Cloud Data Governance and Catalog user, you can specify your rule requirement in plain text: "Customer Identifiers must have eight characters and start with C," and invoke CLAIRE to help. As illustrated in Figure 3, CLAIRE will analyze your requirement using ML and NLP techniques and translate it into a technical representation.
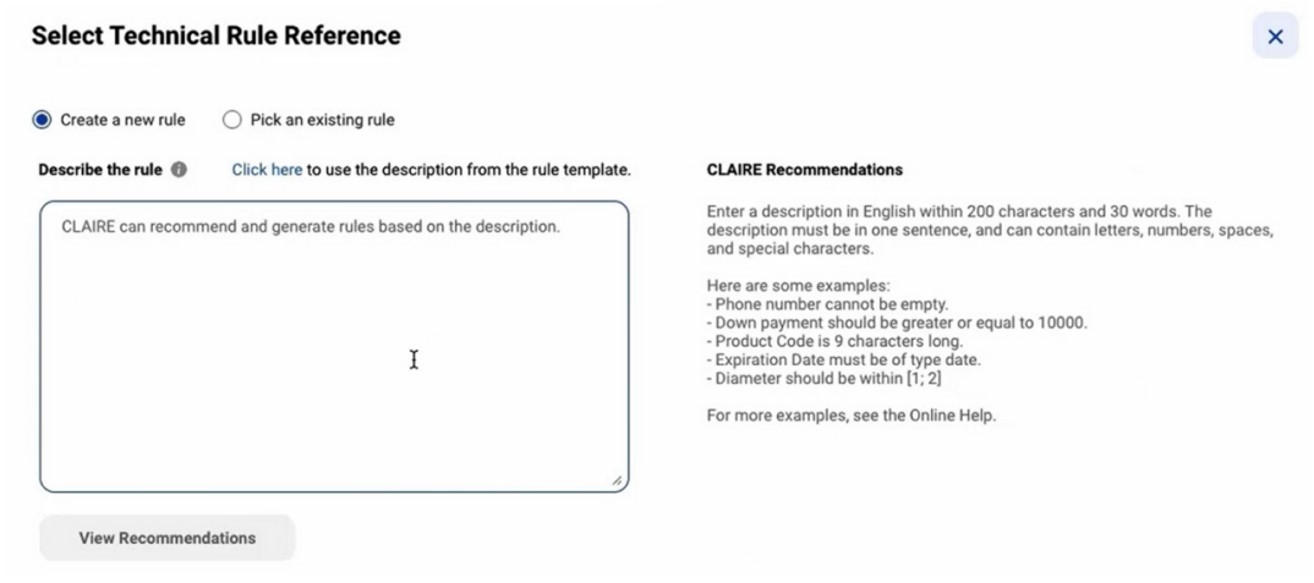
CLAIRE can generate a new data quality rule by using the representation and metadata available, such as the “Glossary Term” name. This rule will fulfill the requirement of the data quality repository and will be linked to the Cloud Data Governance context. Moreover, CLAIRE associates data quality rules to cloud profiles based on the matching column and source object name. By default, data profiling associates rules with columns of Oracle, flat file, ODBC and Amazon S3 V2 connections. As users create new profiles against core objects from one of these sources, CLAIRE will automatically suggest the best-practice data quality rules that should be applied to the measurement.
Intelligent Data Similarity
CLAIRE acts as a copilot using ML techniques like clustering to detect similar data across thousands of databases and file sets. Intelligent data similarity is one of the critical capabilities used for multiple purposes, including identifying data, detecting duplicates, combining individual data fields into business entities, propagating tags across datasets and recommending datasets as a trusted user copilot. Data similarity computes the extent to which data in two columns is the same. A brute-force approach to try and compare all two-column pairs in an enterprise setting (say, across 100 million columns) would be computationally prohibitive. Instead, data similarity uses machine-learning techniques to cluster similar columns and identify likely matches. The process works in multiple stages. First, columns are clustered based on column features. Then, data overlap is computed for unique values in each cluster. Finally, the most promising pairs are chosen for computing data similarity using the Bray-Curtis and Jaccard coefficients. See Figure 4.
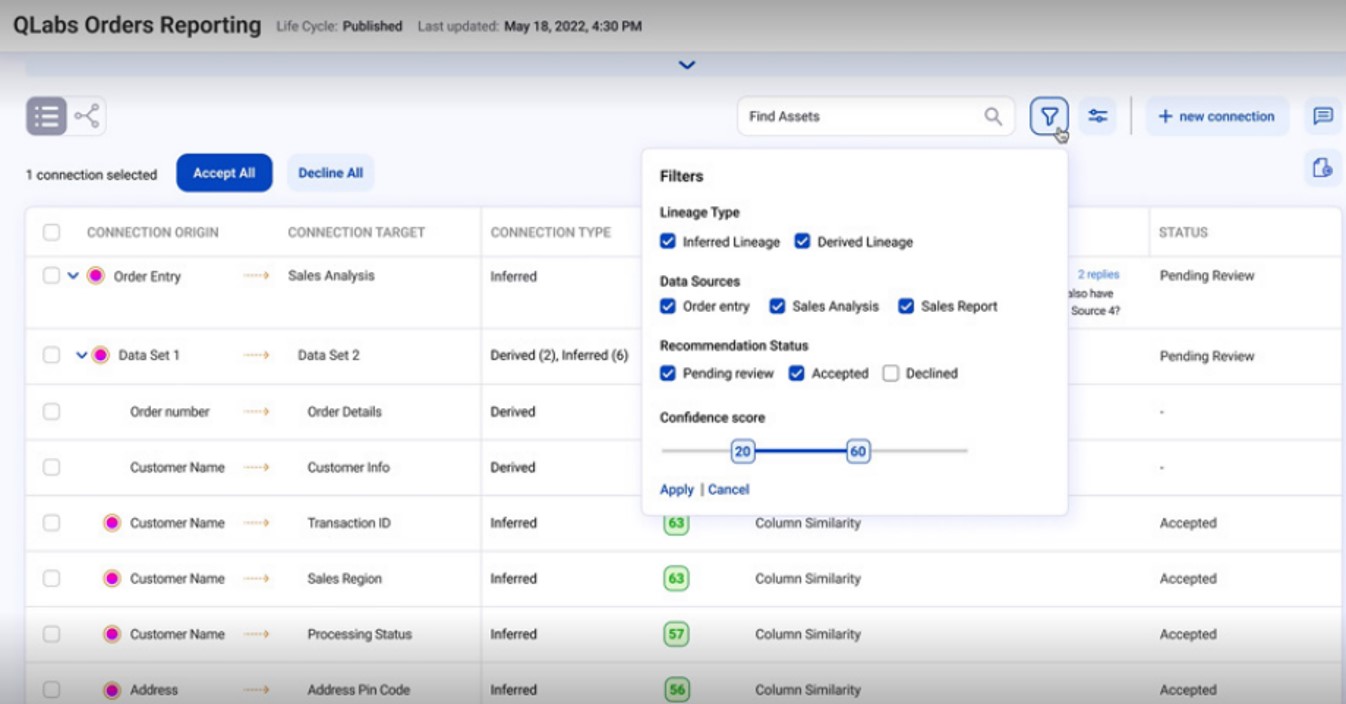
Automatically Associating Business Terms with Physical Datasets
Data governance requires the documentation of business artifacts, definitions, stakeholders, processes, policies and more. To enable a truly aligned view, users must be able to associate definitions and business views to the underlying technical implementations in their data estate. Typically, this task is slow, laborious and error-prone — relying on key people to communicate and manually line up technical manifestations one by one — a task that can take days, weeks or even months to complete. Informatica Cloud Data Governance and Catalog can shortcut this process. With CLAIRE as your copilot, users are provided recommendations of relevant and appropriate data elements to be linked as metadata scans are completed. This cuts down the task of searching for, validating and linking data elements, allowing data stewards and the data governance office to focus on their critical tasks. As implementations progress, the process can be completely automated. See Figure 5.
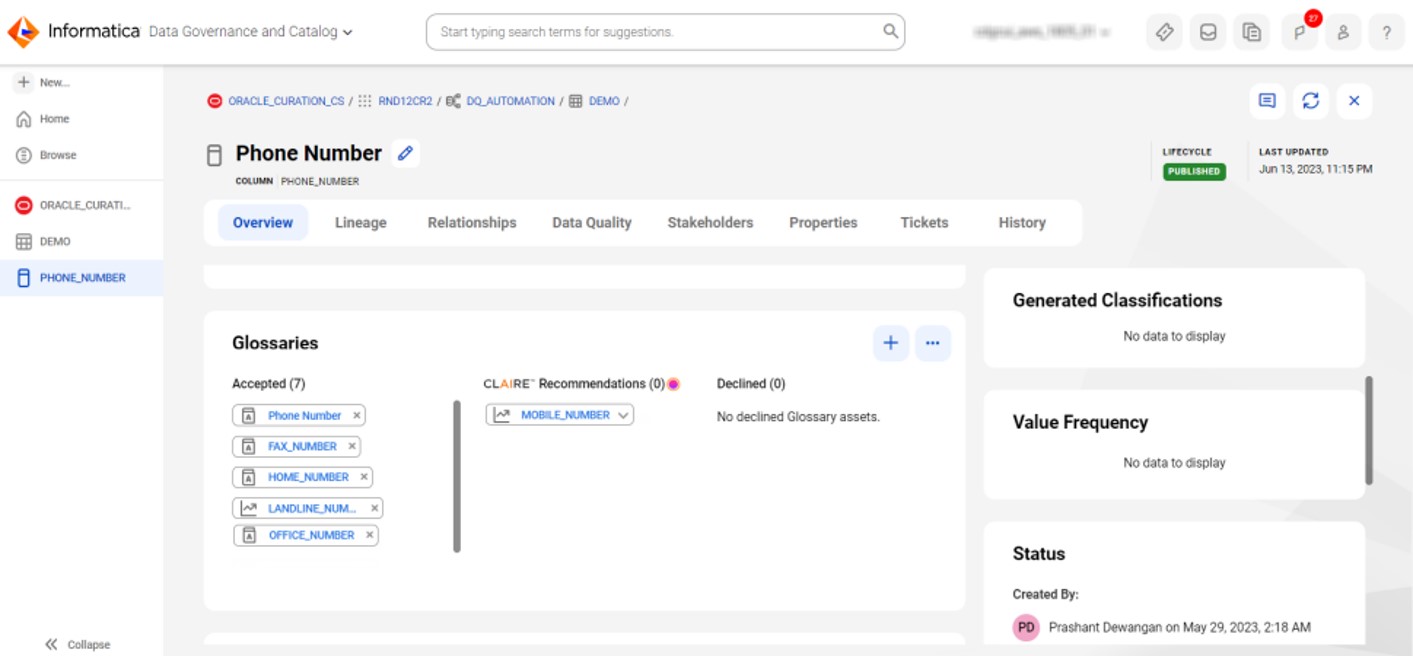
Next Transformation Recommendation
AI revolutionized data integration mapping by automating it and predicting subsequent transformations and expressions. This is achieved by analyzing and utilizing anonymous metadata from the organization's mappings. It generates recommendations for transformations and expressions. Automating repetitive tasks significantly boosts the productivity of data engineers. CLAIRE becomes better with each utilization — acceptance or rejection of the recommendation. Furthermore, it speeds up development by automatically predicting and incorporating the next transformation. See Figure 6.
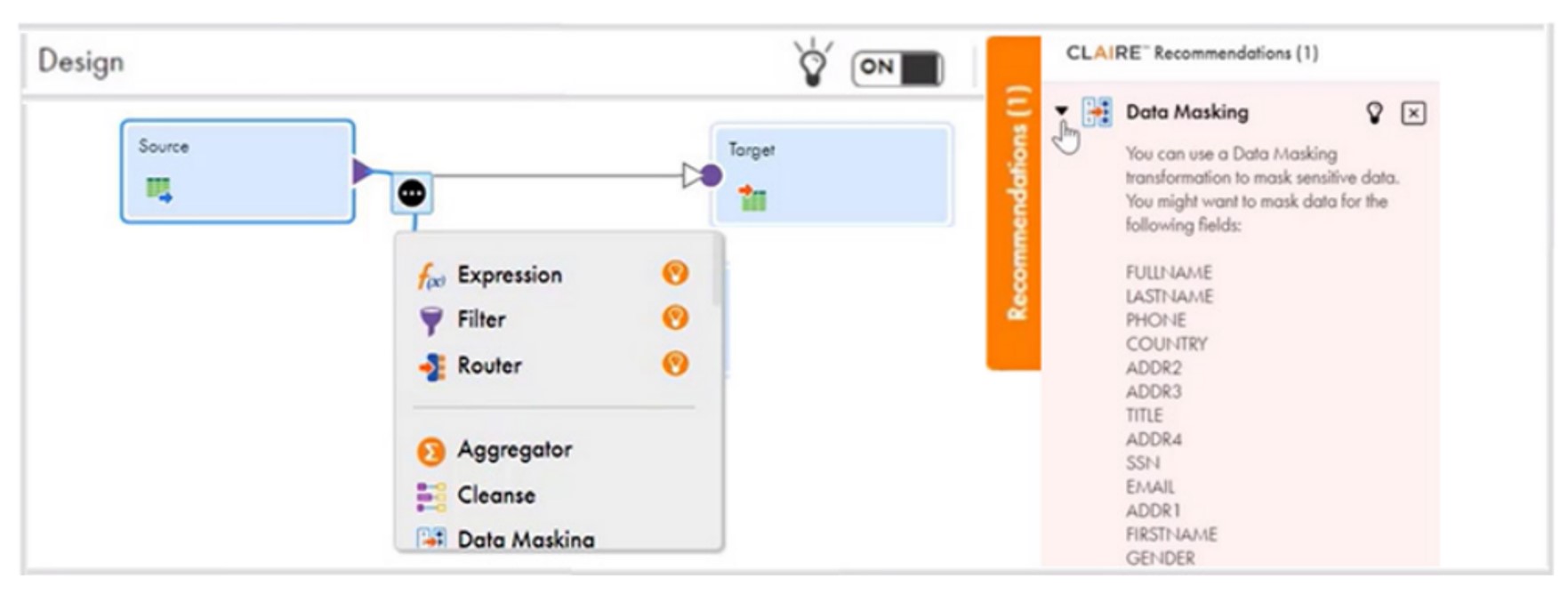
Advanced Relationship Discovery
Discovering relationships is a critical feature that groups similar columns across various data sources, as shown in Figure 7. This technique enhances data governance and boosts business productivity by automatically organizing data. You don't need pre-existing labels to pair similar columns together. The basis for this similarity is the matching of names (metadata), in addition to data match. This intelligent approach significantly reduces expenditures and superfluous costs by eliminating data duplication or redundancy.
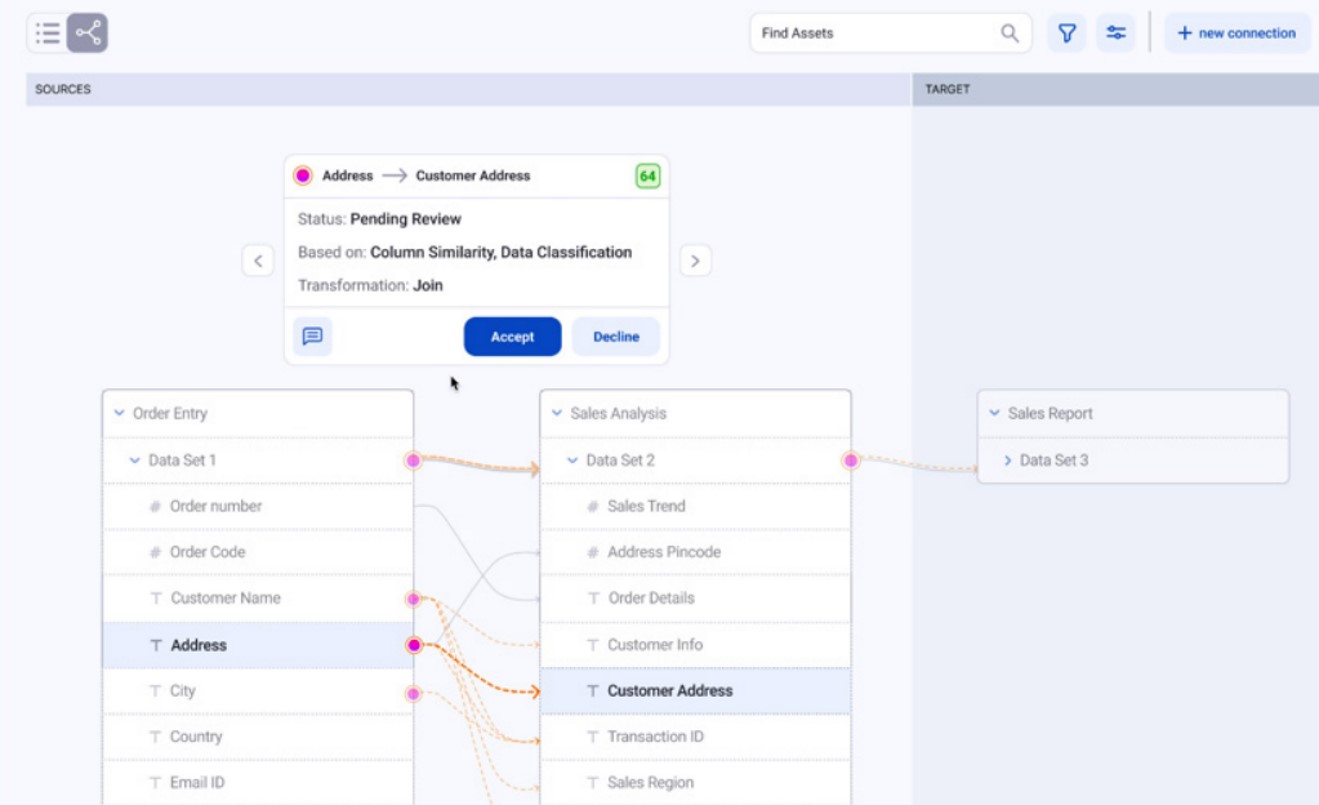
How CLAIRE is Redefining Data Management
The potential of AI in data management doesn't stop with the above scenarios. CLAIRE, with its metadata-driven AI capability, is harnessing the organization's consolidated metadata to automate, scale and add intelligence to routine data management tasks. It is redefining the dynamics of contemporary data management with useful AI/ML capabilities.
Besides providing valuable recommendations and suggesting the next best actions to data engineers, CLAIRE enhances data pipeline performance and preemptively uncovers issues before they disrupt downstream operations. Furthermore, Informatica has integrated comprehensive AI/ML capabilities into the Intelligent Data Management Cloud™ (IDMC), with additional advancements planned.
Learn how our customers benefit from CLAIRE in automating and optimizing data management.
Learn about CLAIRE intelligent matching to create the best-of-breed information about your organization's key business entities.
The Future of CLAIRE Is Here
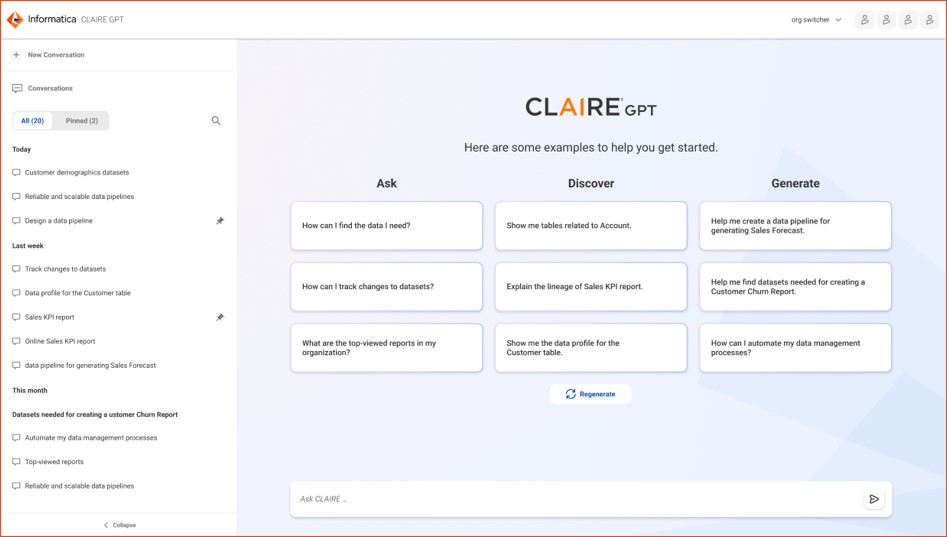
A survey conducted by KPMG found that 77% of business leaders believe that generative AI will have the most significant impact on their businesses out of all emerging technologies. Moreover, 71% of these leaders plan to implement their first generative AI solution within the next two years2.
Informatica is taking a significant leap forward by integrating generative AI into CLAIRE, creating “CLAIRE GPT.” See Figure 8. This service equips data teams with the ability to unlock innovation and productivity. With its intuitive natural language interface, users can ask questions in plain language and receive immediate and actionable insights, streamlining data management for skilled data engineers responsible for creating and supervising their organization's data pipelines. Moreover, it enables non-technical business users to interact with data without SQL knowledge.
Experience the power of CLAIRE through interactive demos.
That’s a Wrap!
Regardless of the size of your enterprise, an AI-driven data management cloud solution can simplify your data stewards, analysts, and scientist's data tasks and facilitate decision-making through intelligent actions and recommendations. CLAIRE is more than just a tool for automating mundane tasks. It's a strategic capability that can provide organizations with a competitive edge. As we move forward, the impact of these next-generation data management solutions will only grow, solidifying their role as indispensable components in the modern data landscape.
Learn more about how CLAIRE has transformed and solved data management challenges.
1 https://www.anaconda.com/resources/whitepapers/state-of-data-science-report-2022/
2 https://kpmg.com/kpmg-us/content/dam/kpmg/pdf/2023/generative-ai-survey.pdf